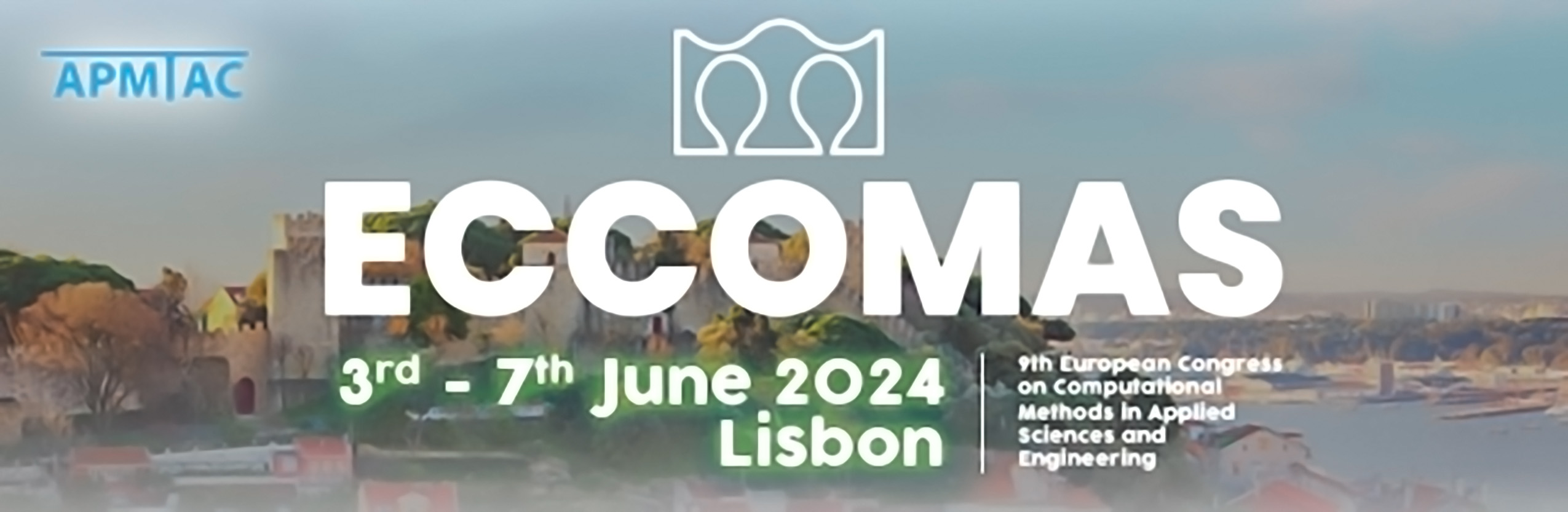
Machine Learning based Approximation of Plastic Deformation during Ditching
Please login to view abstract download link
Simulating dynamic aircraft ditching requires the calculation of hydrodynamic loads, the dynamics and resulting deformations of the aircraft. Usually, dedicated partitioned simulation models, e.g. using FEM (structure) and FVM (multiphase fluid), are used to simulate this challenging fluid-structure interaction problem. Due to the huge associated computational effort, such classical two-way coupling procedures are hardly feasible to optimize the approach conditions and the design in an industrial process. To reduce the computational effort, machine learning (ML) models are combined with advanced efficient fluid dynamic simulation approaches. In previous work, data-driven, order reducing autoencoder based methods were coupled to long short-term memory (LSTM) and Koopman operator strategies to advance the reduced space in time, and have shown great potential to predict ditching loads on a virtually rigid aircraft fuselage [1]. The focus of the presentation is put to the inclusion of approximate plastic deformations using derivatives of these ML procedures. The resulting simulation tool will be applied to a realistic (generic) aircraft ditching in full scale. REFERENCES [1] H. Schwarz, T. Rung and J.-P. M. Zemke, Comparison of LSTM and Koopman-Operator approaches for Predicting Transient Ditching Loads, MORTech 2023 – 6th International Workshop on Model Reduction Techniques, Paris, 2023.