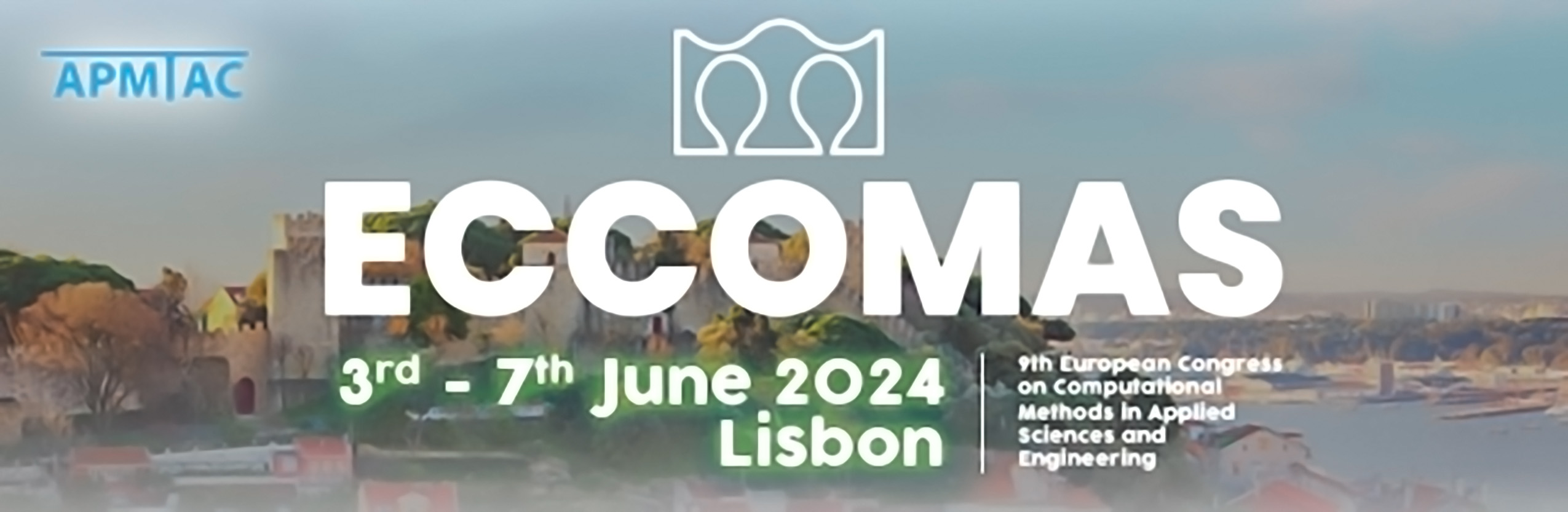
Gaussian Process Learning of Nonlinear Dynamics
Please login to view abstract download link
One of the pivotal tasks in scientific machine learning is to learn the underlying dynamical system with time series data. One of the state-of-the-art methods is sparse identification of non-linear dynamics, leveraging the sparsity promotion to discover the systems. Relevantly, the data-driven operator inference method learns the reduced-order system based on the known physical governing equation. However, learning a dynamical system typically requires estimations of the time derivative of the state data, which is commonly not available and has to be approximated by numerical differentiation. Discrepancy will be therefore introduced when dealing with limited and noisy data. In this work, we propose a data-driven learning method for nonlinear dynamics based on Gaussian process regression. The method leverages the intrinsic closure property of Gaussian process to derive the time derivative from state data and constructs the joint distribution between state data and its time derivatives as the likelihood function in the Bayesian framework. Together with the prior constraint on parametrization, the underlying system can be inferred through Bayesian inference and subsequently make predictions with the quantified uncertainty from data. We present the method's effectiveness through numerical examples in two major dynamics learning scenarios, 1) inference for given parametrization and 2) parametric approximation without any pre-knowledge. The proposed method stands out for its robust compatibility in dealing with scarce and/or noisy data.