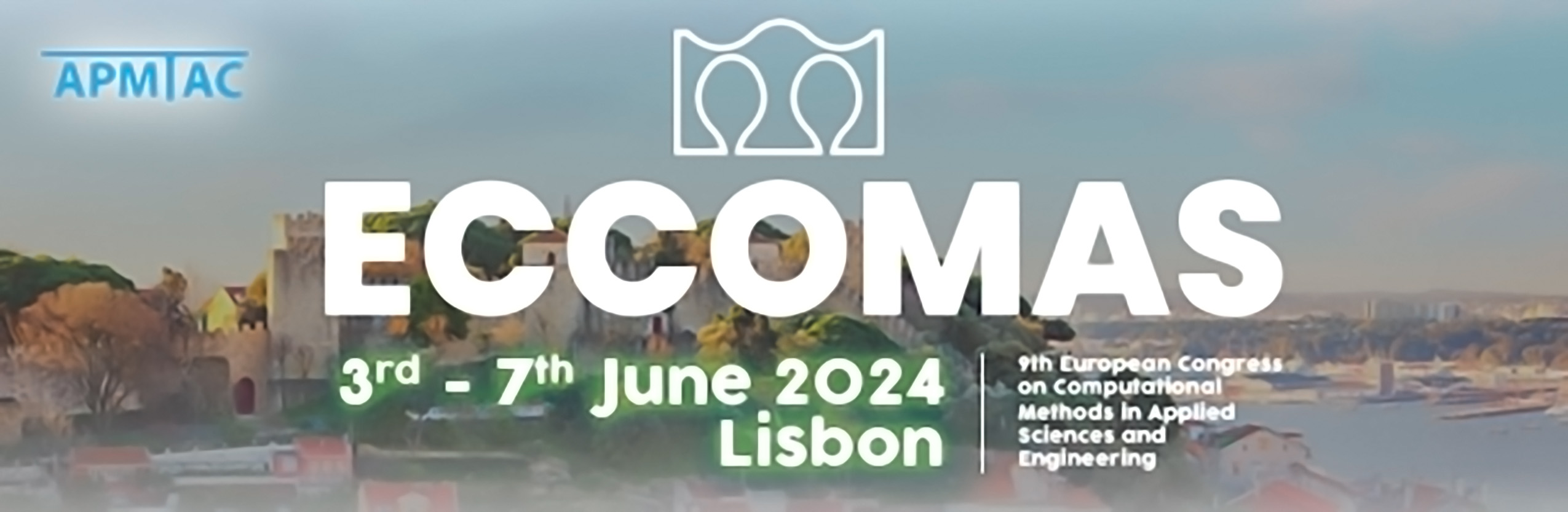
Statistical Learning and Inference in the Small Data and Poor Model Limits
Please login to view abstract download link
We present an exploration of statistical learning for problems with scarce data and insufficient models. The technical challenge resides in extracting sufficient constraints from limited available data, supplement it with physics-informed insight, to deduce credible predictions concerning relevant qualities of interest. We demonstrate and compare a number of statistical learning frameworks including Gaussian processes (GP), Probabilistic Leaning on Manifolds (PLoM), and Switching Diffusions (SwD) using experimental data pertaining to materials science, reactive flows, and sovereign ratings. The GP approach is an example of supervised learning with statistical context. PLoM is an example of unsupervised learning where joint density functions are estimated around an intrinsic structure characterized by diffusion coordinates. SwD is an example of coupled chains representing physics insight concerning multiscale interactions. The diversity of the applications explored in our presentation, ranging from physical sciences and engineering to economics tests the validity of the mathematical and statistical constructs underpinning these methods. We explore the applicability and accuracy of these methods for the various applications, highlighting the effect of data and model errors on their suitability.