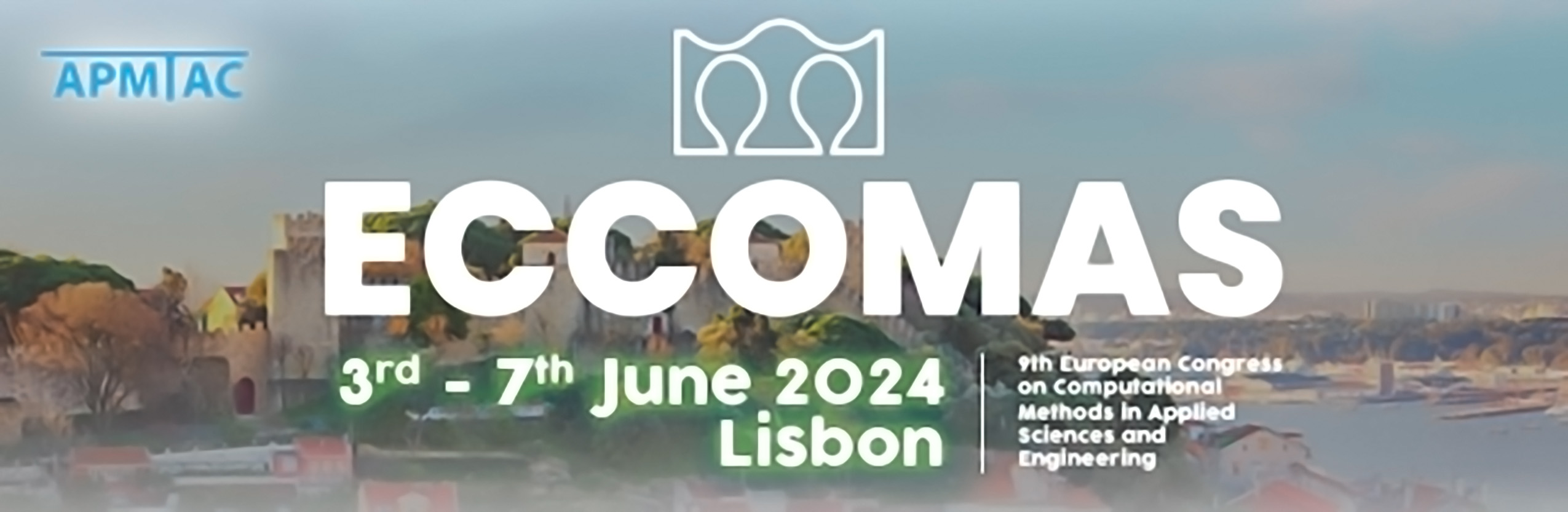
Theoretical Insights of Practical Interest for Consensus-Based Optimization
Please login to view abstract download link
Consensus-based optimization (CBO) is a multi-agent derivative-free optimization method capable of globally minimizing high-dimensional nonconvex and nonsmooth functions, a problem which is at the very foundations of science and engineering, machine learning, and beyond. With this talk we provide insights into the internal mechanisms of CBO, which are responsible for the success of the method. First, based on an experimentally supported intuition that, in the mean-field limit (as the number of particles goes to infinity), CBO always performs a gradient descent of the squared Euclidean distance to the global minimizer, we develop a novel technique for proving global convergence in mean-field law for a rich class of objective functions. In particular, we show that CBO performs a convexification of a very large class of optimization problems as the number of optimizing particles tends to infinity. From this result it becomes apparent that the hardness of any global optimization problem is necessarily encoded in the mean-field approximation, for which we present a probabilistic quantitative result. A combination of these results allows to obtain a holistic convergence proof of CBO methods on the plane. Secondly, by changing perspective towards the end of the talk and turning our back on the previous mean-field-focused analysis point of view, we discover an intriguing connection between CBO and gradient flows. We interpret CBO as a stochastic relaxation of gradient descent, thereby providing a novel analytical perspective on the theoretical understanding of gradient-based learning algorithms. We observe that through communication of the particles, CBO exhibits a stochastic gradient descent (SGD)-like behavior despite solely relying on evaluations of the objective function. The fundamental value of such link between CBO and SGD lies in the formerly established fact that CBO is provably globally convergent to global minimizers, hence, on the one side, offering a novel explanation for the success of stochastic relaxations of gradient descent, and, on the other side and contrary to the conventional wisdom for which zero-order methods ought to be inefficient or not to possess generalization abilities, unveiling an intrinsic gradient descent nature of such heuristics. With this we furnish insights that explain how stochastic perturbations of gradient descent overcome energy barriers and reach deep levels of nonconvex functions.