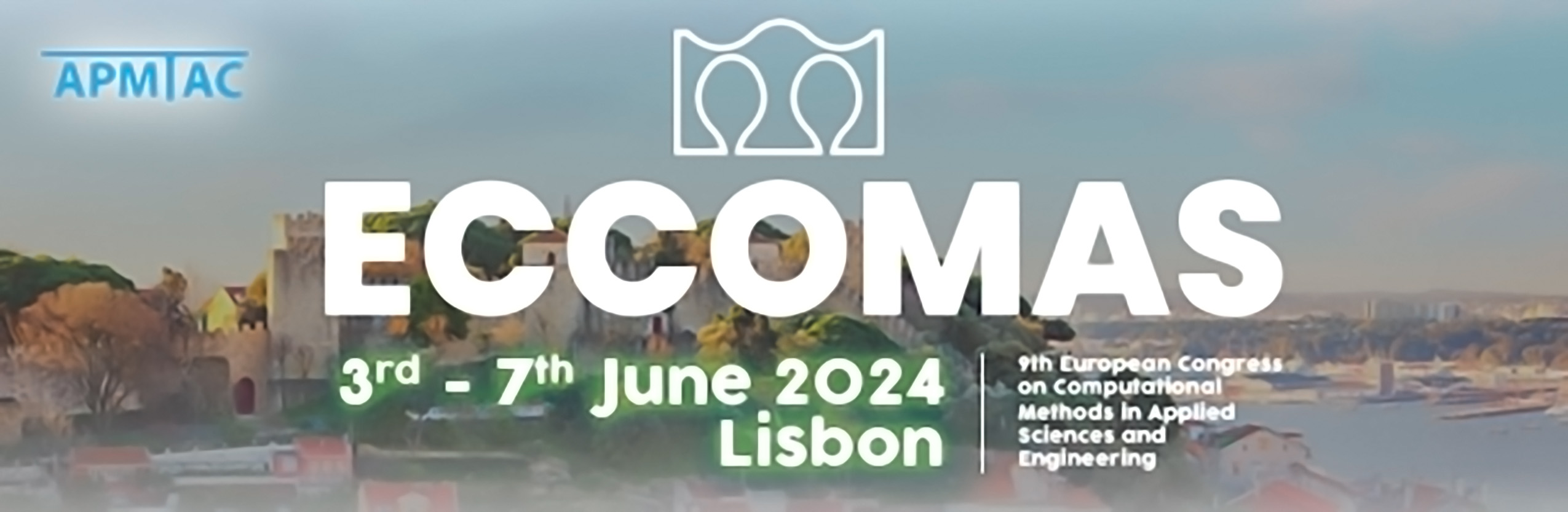
Goal-Oriented Adaptivity for solving Partial Differential Equations using Neural Networks
Please login to view abstract download link
Over the last two decades, Goal-Oriented Adaptivity (GOA) has been extensively studied and developed for the Finite Element Method (FEM). It is a technique that consists of enhancing the discretization of the underlying mesh with the aim of approximating a specific Quantity of Interest (QoI) rather than the error in the energy norm. In this work, instead of considering a FEM discretization, we use neural networks. By adopting an Extreme Learning Machine interpretation for feed-forward neural networks, we are able to establish a Petrov-Galerkin-type approach such that: (i) the basis functions are no longer locally supported, and thus possess the potential to overcome the curse of dimensionality; (ii) the basis functions are governed by trainable parameters, yielding a highly non-linear scheme; and (iii) for each choice of trainable parameters, the optimal coefficients of the corresponding linear combination are efficiently computable following a minimum residual methodology. In this way, each GOA iteration is identified in our proposal as a training step of neural networks where the loss function represents an appropriate upper bound of the QoI. We restrict ourselves to symmetric and positive-definite problems to ensure that we can incorporate robust error estimators for the primal and dual problems within the loss function. Extending it to non-symmetric or indefinite problems requires special care, which we plan to address in future work. The numerical experiments conducted in different spatial dimensions demonstrate the effectiveness of our strategy, up to the optimizer performance capacity and the usual numerical integration challenges.