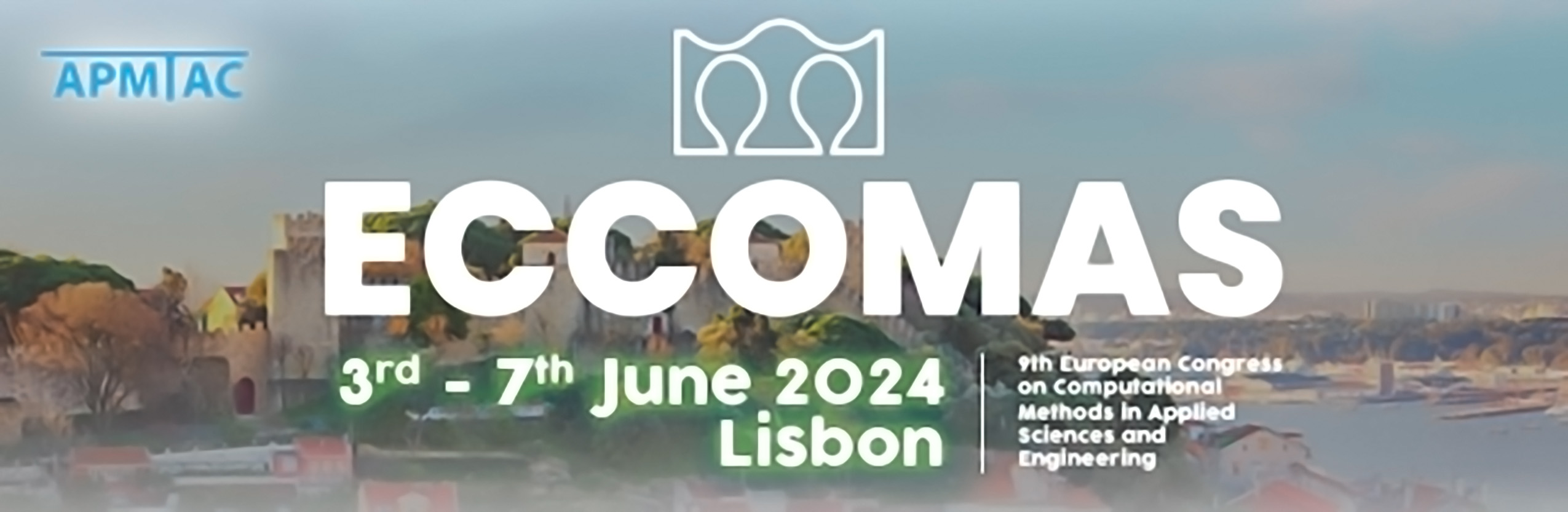
Structural Stress Estimation using Digital Image Correlation and Machine Learning
Please login to view abstract download link
Four main features of Structural Health Monitoring (SHM) processes are: (a) damage detection, (b) damage localization, (c) damage classification, and (d) determining remaining life of the structure. The following contribution focuses on the last feature that is also referred to as Operational Load Monitoring (OLM) or fatigue tracking. It consists of measuring and registering the number and amplitude of load cycles that a structure has withstood in order to plan maintenance actions before fatigue failure happens . Machine learning techniques have revolutionized SHM, including OLM, processes. In typical OLM approach data from strain sensors is processed by a trained prediction model to obtain information about current load or stress of the structure. In the following contribution a different approach is proposed where digital image correlation (DIC) is used instead of point measurements, where only a relatively small fragment of the structure is observed. For this fragment a full strain/displacement map can be obtained. Current state of the whole structure can be estimated using a carefully trained prediction model. The input to the prediction model are the values from the DIC strain/displacement maps. An example is provided where hat-stiffened composite panel, a typical structure used in modern aerospace industry, is subjected to different loads in an experimental setup. Only a small part of the structure is monitored using DIC. A neural network is trained to process the DIC results for structural stress estimation.