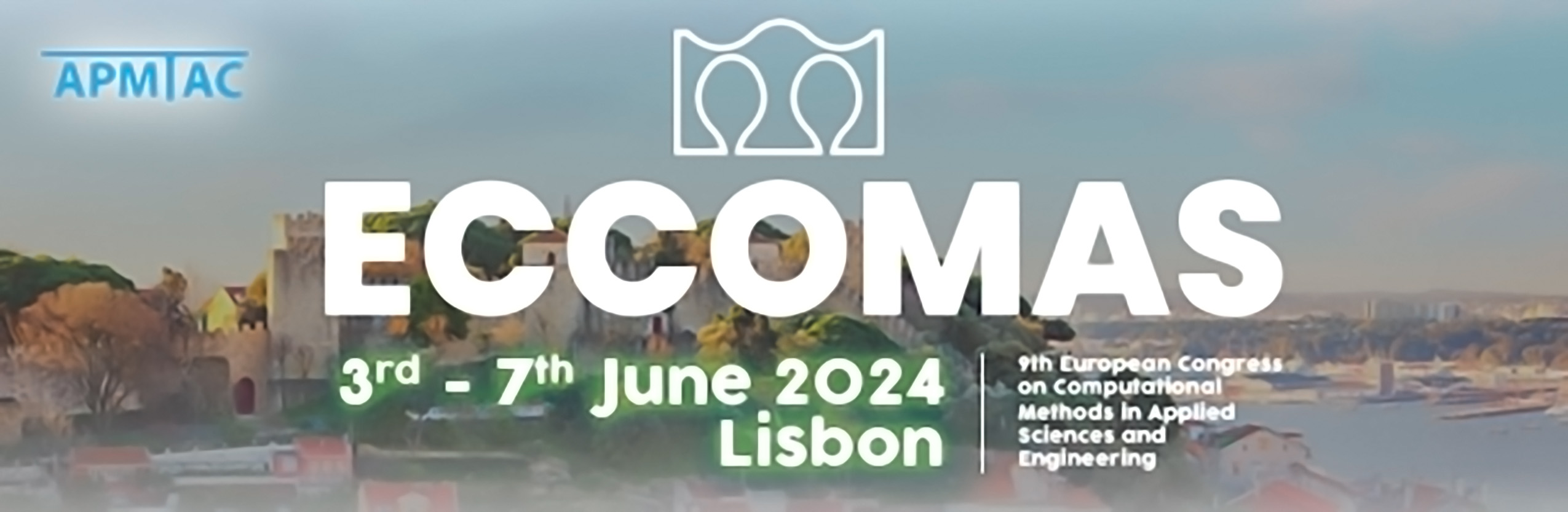
LaSDI: Latent space dynamics identification
Please login to view abstract download link
A family of latent space dynamics identification (LaSDI) frameworks will be introduced, i.e., LaSDI [1], gLaSDI [2], GPLaSDI [3], WLaSDI [4], and tLaSDI. LaSDI is an in- terpretable data-driven framework that follows three distinct steps, i.e., compression, dynamics identification, and prediction. Compression allows high-dimensional data to be reduced so that they can be easily fit into an interpretable model. Dynamics iden- tification lets you derive the interpretable model, usually some form of parameterized differential equations that fit the reduced latent space data. Then, in the prediction phase, the identified differential equations are solved in the reduced space for a new pa- rameter point and its solution is projected back to the full space. The efficiency of the LaSDI framework comes from the fact that the solution process in the prediction phase does not involve any full order model size. For the identification, various approaches are possible, e.g., a fixed form as in dynamic mode decomposition and thermodynamics-based LaSDI, a regression form as in sparse identification of nonlinear dynamics (SINDy) [5] and weak SINDy [6], and a physics-driven form as projection-based reduced order model [7]. Various physics problems were accurately accelerated by the family of LaSDIs, achieving aspeed-up of 1000x, e.g., kinetic plasma simulations [10], advanced manufacturing process simulations [9], pore collapse [8], and transport equations.