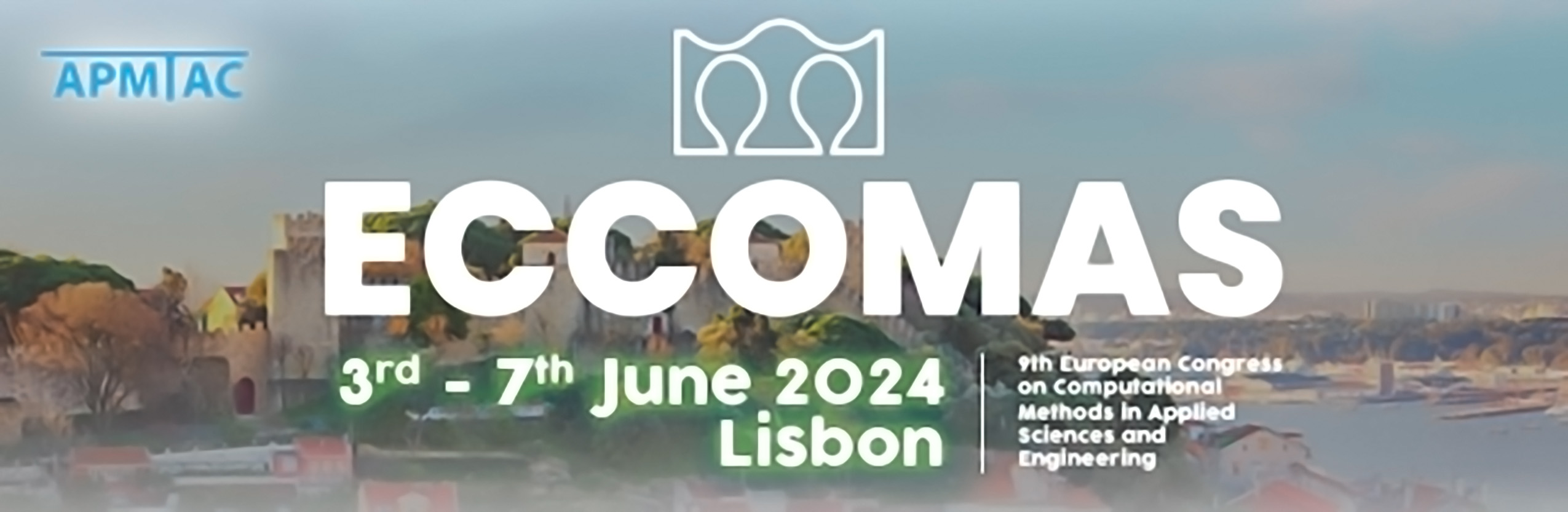
Progressive augmentation of RANS turbulence models by simulation-driven surrogate optimisation
Please login to view abstract download link
The consistency of the a posteriori results and generalisability are the most critical aspects of new data-driven turbulence models. The generalisability challenge extends beyond addressing unseen cases outside the training dataset; it also involves the performance of new models on canonical cases such as channel flow. This study presents the progressive enhancement of the $k-\omega$ SST model for its known shortcomings in the prediction of the secondary flows of the second kind and the flow separation. The progressive approach is also combined with a simulation-driven optimisation technique to ensure the consistency of the a posteriori results. In the first part of the study, the k-omega SST model is enhanced with the capability of predicting Pradtl's second kind of secondary flow without violating its original performance on canonical cases e.g. channel flow and periodic hills. The optimisation is based on a case square-duct flow to solely obtain an optimised correction for the secondary-flow effect. In the second part, a separation factor is introduced in the k-omega SST model to modify the turbulent viscosity while ensuring its activation only in the case of flow separation. The separation factor is optimised based on its performance in multiple training cases including periodic hills and curved backward-facing step flow. Regarding their generalisability, the new models are tested on unseen cases with different geometries and Reynolds numbers, showing a successful improvement in the prediction of Pradtl's second kind of secondary flows and flow separation. These findings highlight the capability of the progressive approach to enhance the performance of linear eddy-viscosity turbulence models with data-driven methods while preserving the robustness and stability of the original models.