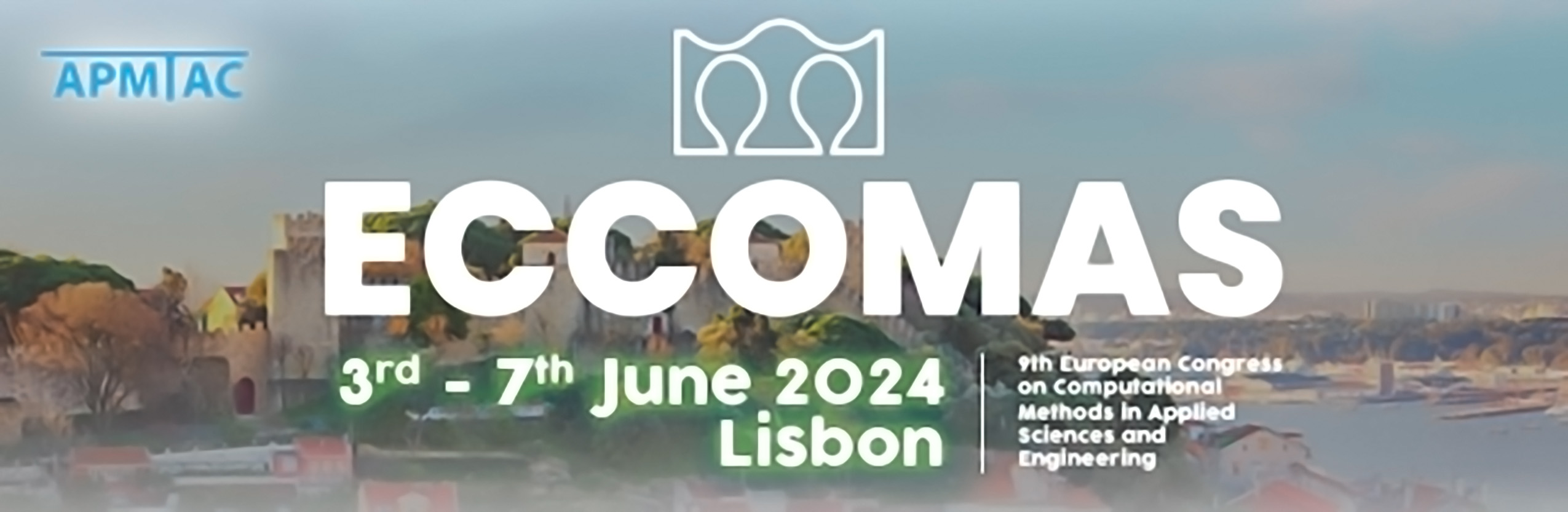
Physically enhanced neural networks: Application to nonlinear viscoelasticity
Please login to view abstract download link
Modern neural networks are known for their ability to process large data sets and recognize relationships between different variables. While they are traditionally used in data-centric applications, in recent years there has been a surge of interest in using neural networks for physically motivated problems. Classical feed-forward neural networks are excellent at interpolation, but often fail at extrapolation, especially when confronted with noisy experimental data. With a focus on material modeling, this paper deals with the intricacies of physically motivated neural network methods. It explores the optimal selection of input and output variables, while taking into account fundamental physical axioms, such as the non-negativity of the strain energy function. In addition, the use of invariants as input variables is analyzed. The discussion extends to the construction and training of neural networks and demonstrates their effectiveness in creating elastic, hyperelastic and viscoelastic material models with limited data. In order to demonstrate the practical applicability, the trained networks are integrated into a three-dimensional finite element code. Comparative analyses with classical material models will be performed to evaluate the accuracy and computational efficiency. This investigation serves to illustrate both the potential and challenges associated with the integration of physically augmented neural networks into the field of advanced material modeling.