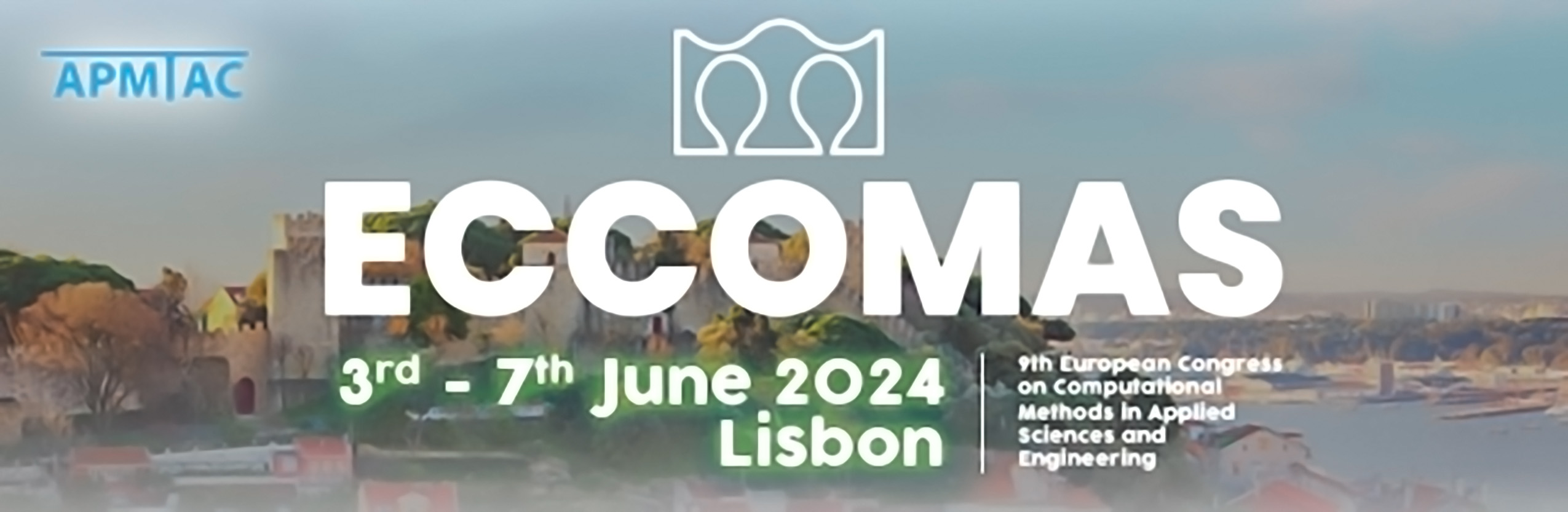
Accounting for elasto-plasticity in constitutive artificial neural networks
Please login to view abstract download link
Constantly advancing technologies in manufacturing processes enable the development of new materials. In order to accurately predict their material behaviour, new types of material models and combinations of existing, sophisticated models must be developed. To date, there have been a large number of constitutive models derived from the principles of thermodynamics. For innovative material combinations, however, the question arises as to which constitutive model should be chosen and how a new, accurate model can be formulated. To facilitate the search for suitable new models, in this work, we use data-driven models using machine learning to predict mechanical behaviour through constitutive artificial neural networks (CANN). Promising results for elastic material behaviour have already been described by Linka et al. [1], and an extension to viscoelastic behaviour was recently published by Holthusen et al. [2]. However, the extension to elasto-plastic material behaviour is still open. Therefore, we propose a novel formulation of a constitutive artificial neural network based on the above work that can be applied to elasto-plasticity by combining feed-forward networks of Helmholtz free energy and yield function. Physical information such as stress-strain data and conditions from material theory are included so that the resulting physically informed CANN leads directly to constitutive models that fulfil the principles of thermodynamics a priori. As a result, this work introduces a novel formulation of a constitutive artificial neural network that captures elasto-plastic material behaviour. REFERENCES [1] K Linka, M Hillgärtner, KP Abdolazizi, RC Aydin, M Itskov, CJ Cyron: Constitutive artificial neural networks: A fast and general approach to predictive data-driven constitutive modeling by deep learning. Journal of Computational Physics 429: 110010, 2021. [2] H Holthusen, L Lamm, T Brepols, S Reese, E Kuhl: Theory and implementation of inelastic Constitutive Artificial Neural Networks. arXiv preprint arXiv:2311.06380, 2023.