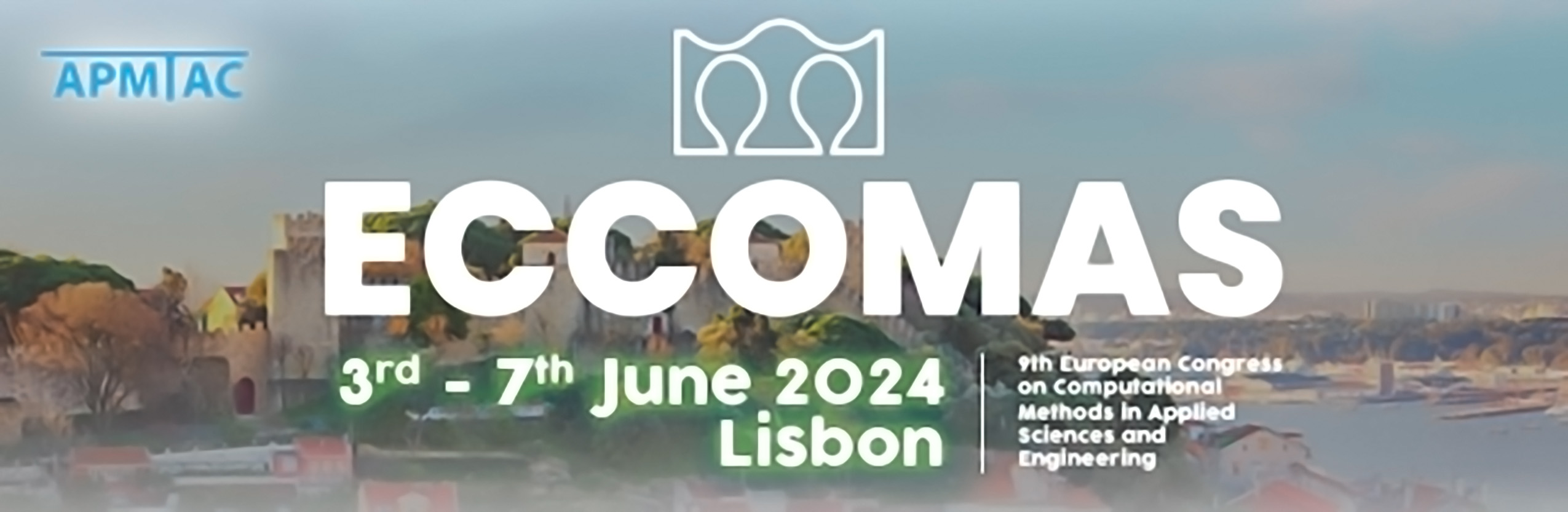
The Interplay of Model Form, Uncertainty Quantification and Error in Computational Predictions from a Validation Assessment Perspective
Please login to view abstract download link
Knowledge of the accuracy and uncertainty in computational prediction is paramount for informed-decision-making. Such knowledge is also needed for industrial process modeling to ensure product quality, design, and many other applications where simulations are used in both science and engineering. The credibility case for a given intended use of a computational simulation depends on the underlying model, its numeric implementation, the data used to calibrate the model and the data used to validate the simulation capability for the questions of interest (QoI) and their associated metrics. Here model is used in the general sense--mathematical, physics-based, engineering-based, or data-driven are all included. This talk uses a simple example to demonstrate the interplay of quantification of uncertainties, model form and error in providing the credibility case for using results from computational simulations. De facto, this also defines “credibility”. The goal is to provide a simple understanding of the considerations needed to assess credibility, including: evaluation of conditions for intended use (and appropriate use); comparison of interpolation and extrapolation; and the role of model form discrepancy together with uncertainty quantification has on “prediction”.