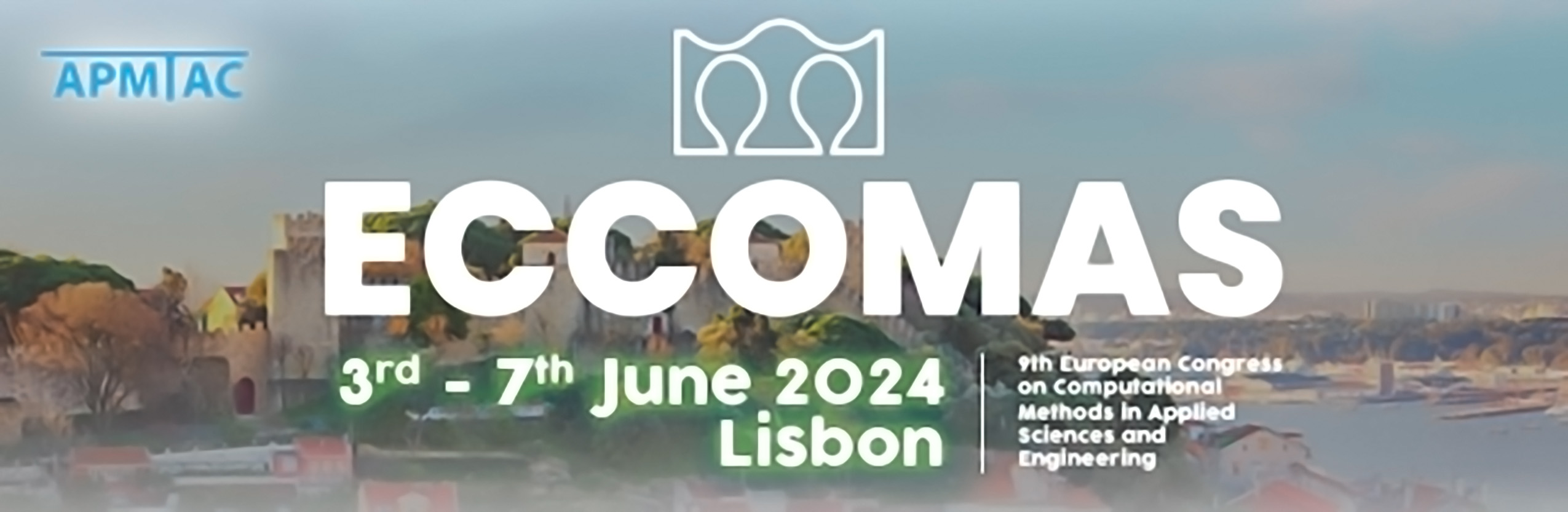
Trajectory optimization of undulatory swimmers using model predictive control
Please login to view abstract download link
The motion of self-propelled undulatory swimmers is governed by complex nonlinear dynamical systems resulting from a two-way interaction between the swimmer and the surrounding fluid. Learning and understanding these dynamical systems constitute pivotal initial steps toward the precise control of swimming behavior. In this study, we explore a model-based approach by combining computational fluid dynamics (CFD) simulations with modern machine learning techniques. Our strategy involves utilizing data generated from CFD simulations to perform nonlinear system identification. The sparse identification of nonlinear dynamics algorithm (sindy) is utilized to learn the models, which subsequently serve as a foundation for the development of efficient control strategies. Our research addresses two distinct control challenges. Firstly, we employ model predictive control to enable precise velocity tracking. Secondly, we use an iterative learning control strategy to optimize the swimmer’s efficiency. By delving into these control problems, we aim to shed light on the intricate interplay between fluid dynamics and swimming kinematics, ultimately contributing to advancements in the control and manipulation of aquatic locomotion.