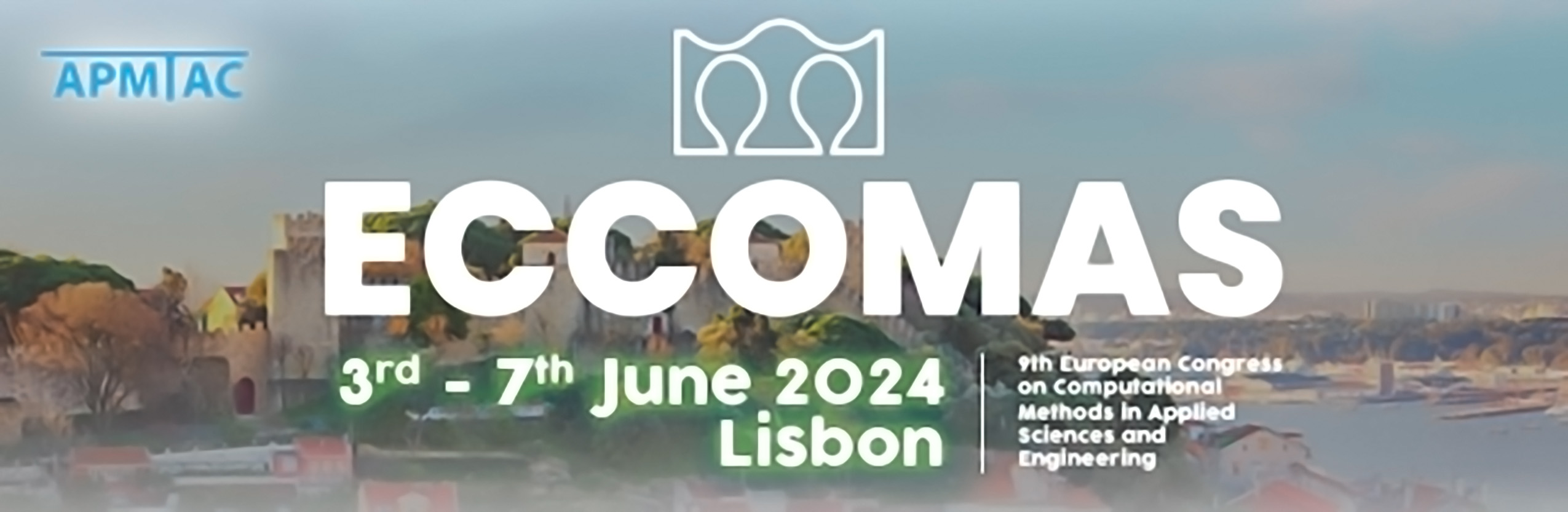
Towards Active Flow Control Strategies Through Deep Reinforcement Learning
Please login to view abstract download link
The present work proposes to explore deep reinforcement learning (DRL) methods for designing optimal active flow control (AFC) strategies for drag reduction using synthetic jets in wings. In the present study, the SD7003 airfoil is investigated at Re=60,000 and considering two angles of attack, 4º and 14º. Therefore, the capabilities of combining DRL and AFC in reducing the drag coefficient in wings is assessed for both, wall-bounded and massive separated conditions, respectively.