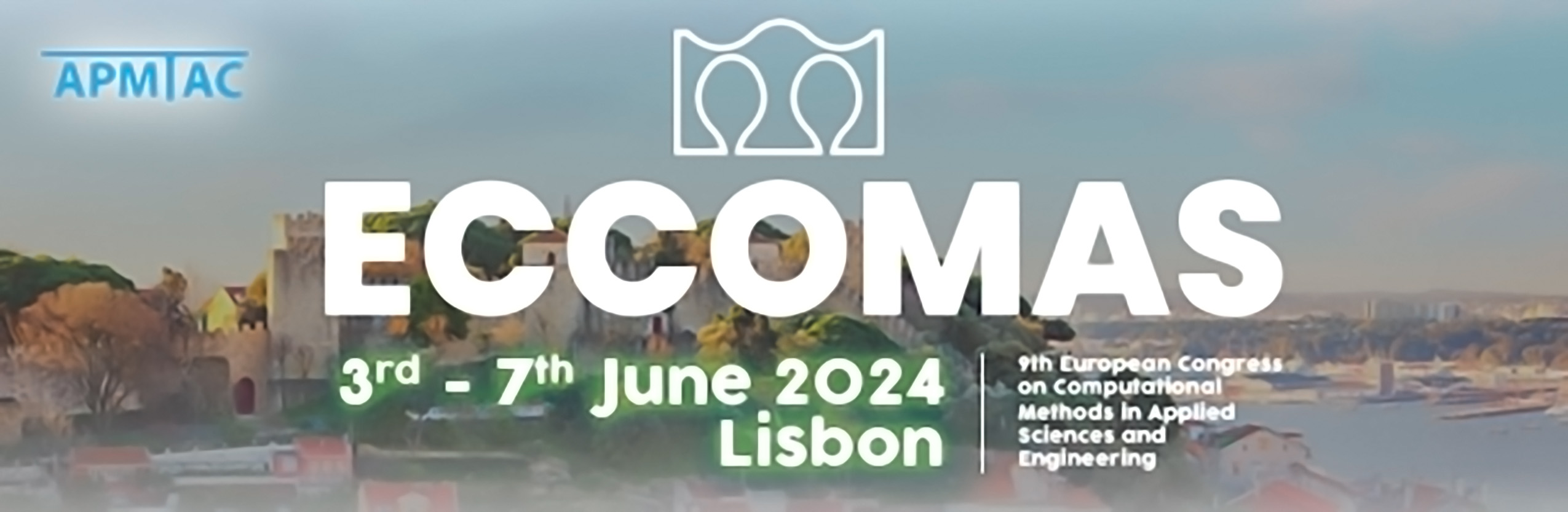
Efficient Multiscale Analysis of Woven Composites Using Physics-based Hierarchical Neural Networks
Please login to view abstract download link
Multiscale finite element analysis of woven composites often requires detailed micromechanical evaluations, leading to significant computational demands. Data-driven surrogate models have been used to overcome the computational burden of coupled multi-scale models. The present study offers a solution using neural network surrogate models specifically designed to overcome the limitations of traditional data-driven networks in terms of interpretability and extrapolation. We propose incorporating physics-based constitutive material models as activation functions in a neural network's latent space based on recent developments by \cite{Maia}, enabling path-dependent behavior recognition. To expand the method capabilities, a hierarchical multiscale physics-based neural network model is developed to quickly and accurately predict the homogenized elasto-plastic behavior of a mesoscale woven composite's representative volume element. A hybrid neural network with physics-based elasto-plastic constitutive models is trained at the microscale. The trained network is used as the fictitious material point surrogate at the upper scale to train a second network at the mesoscale. %while the parameters of the first network are frozen at this point. Constitutive model parameters are updated by the neural network based on strains at the upper scale, while the constitutive model edits internal parameters governing the plastic strain flow rule. Different load cases at micro- and mesoscale are considered to validate the approach. Physics-based hierarchical neural networks have training time advantages over conventional recurrent neural networks due to their straightforward unit structures. Meanwhile, the results of this study demonstrate that the trained networks are capable of predicting both micro- and mesoscale composite behavior from a limited number of loading scenarios.