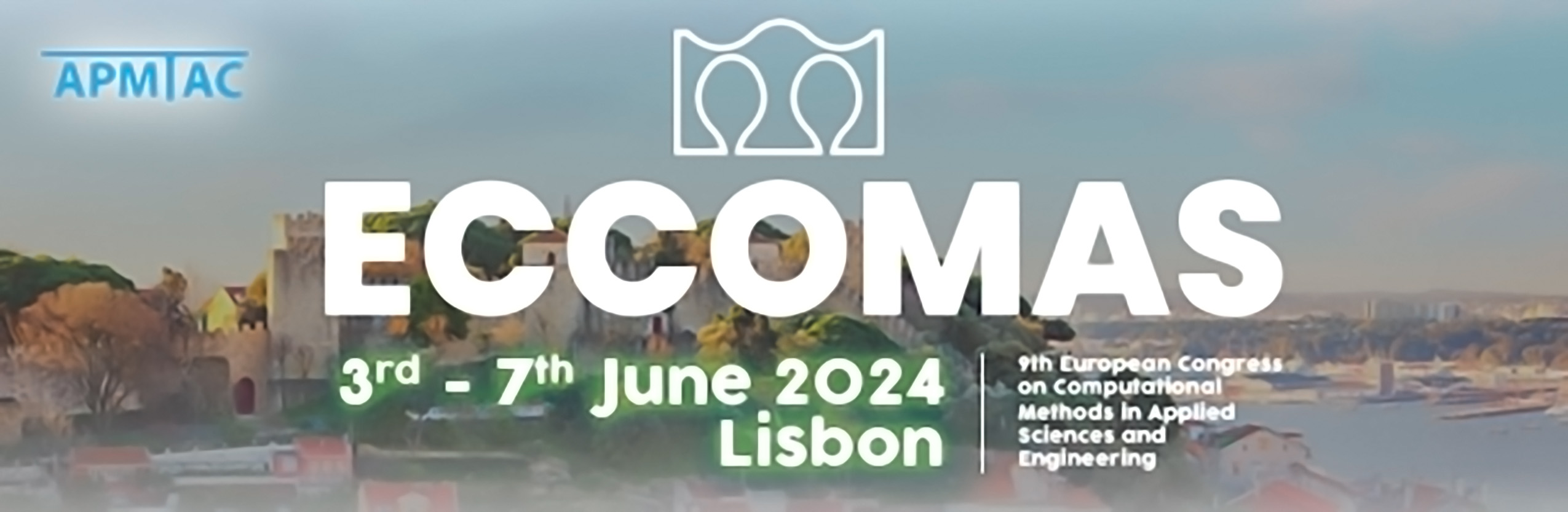
Automotive motor rotor design synthesis using conditional Wasserstein Generative Adversarial Networks with gradient penalty and distortion penalty
Please login to view abstract download link
This study proposes a motor-rotor-shape generation method based on a conditional Wasserstein generative adversarial networks (GAN) with gradient penalty (cWGAN-gp). In the literature, several studies on rotor generation employing the GAN model utilize images as input data[1][2]. In these studies, the GAN output remains limited because the training data is represented by a set of parameters, or the GAN output contains gray-scale area and outlines of the shape are not clear. In this study, the training data was composed of a sequence of points to generate motor shape with a high degree of freedom. Further, we introduced a distortion degree as a regularization in the training process to generate rotor shapes that are smooth, intuitively understandable, and easy to manufacture. Rotor shapes generated by cWGAN-gp indicated characteristics similar to a multiplication of training data with performances close to that of input label data. Furthermore, Smooth shapes were generated by properly setting the influence of the distortion degree. The motor shapes could be generated accurately when a sufficient amount of training data existed. These results show that by training the model with the accumulated motor data, new shapes based on the input performance can be generated quickly. This makes it possible to propose multiple rotor shapes with various performances in a short period of time in the early stages of motor design.