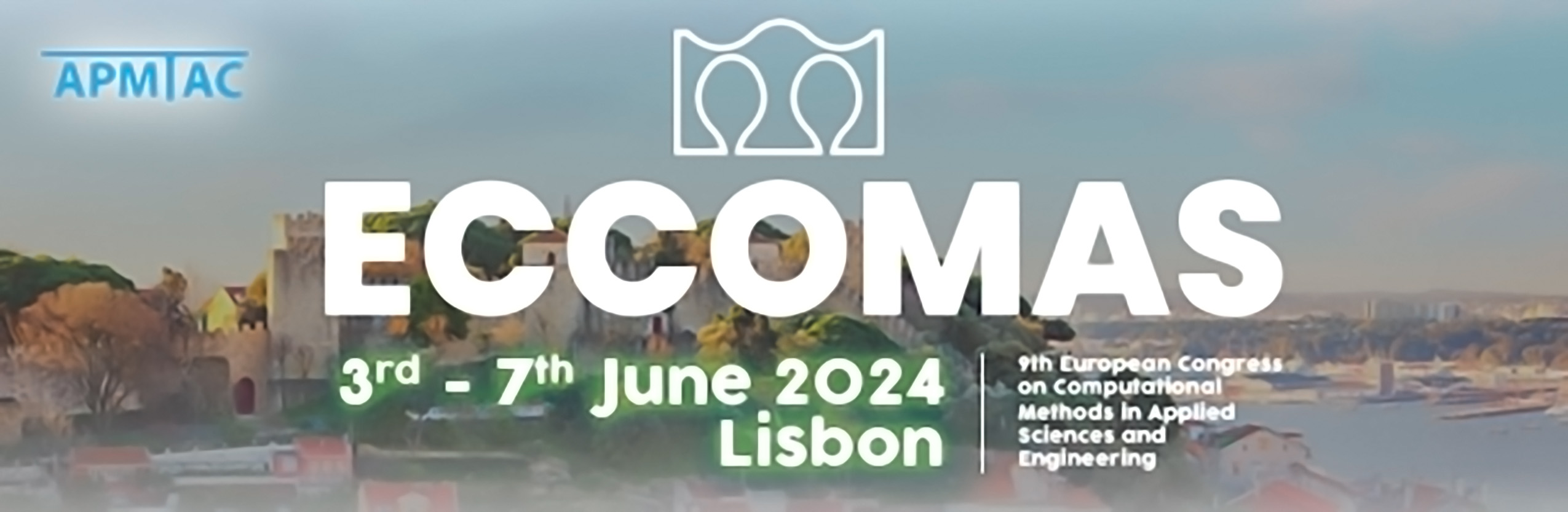
Iterative algorithms for partitioned neural network approximation to partial differential equations
Please login to view abstract download link
Partitioned neural networks have an advantage over the standard single neural network approximation in that they allow more flexible hyper parameter settings, such as, the number of layers and nodes in the neural networks, the selection of training data sets, the loss function design, and parameter optimization schemes. In addition, the partitioned neural networks are easily adoptable for the parallel computation. In this talk, we present iterative algorithms for partitioned neural network approximation by classical domain decomposition methods. The use the iterative algorithms can greatly reduce the communication cost compared to the epoch-based parameter training schemes and provides a better parallel computing performance. Numerical results are presented for various test examples to show the promising features of the proposed algorithms.