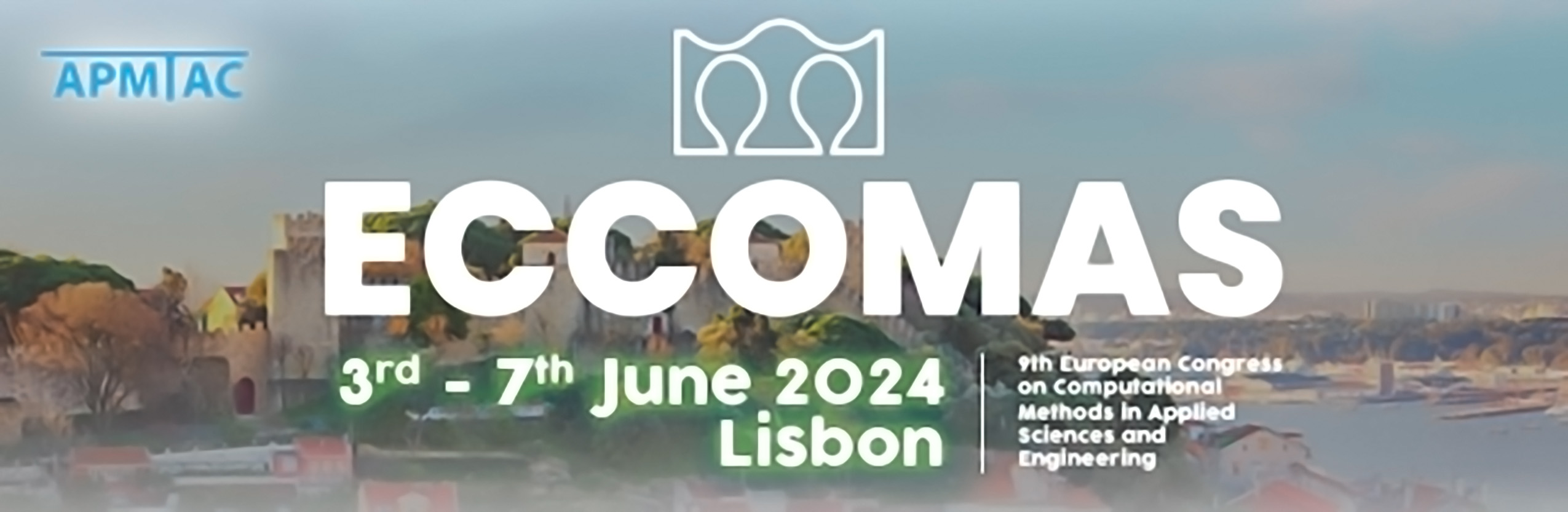
Fast and Reliable Reduced-Order Models for Cardiac Electrophysiology
Please login to view abstract download link
Mathematical models of the human heart increasingly play a vital role in understanding the working mechanisms of the heart, both under healthy functioning and during disease. The ultimate aim is to aid medical practitioners diagnose and treat the many ailments affecting the heart. Towards this, modelling cardiac electrophysiology is crucial as the heart's electrical activity underlies the contraction mechanism and the resulting pumping action. Apart from modelling attempts, the pursuit of efficient, reliable, and fast solution algorithms has been of great importance in this context. The governing equations and the constitutive laws describing the electrical activity in the heart are coupled, nonlinear, and involve a fast moving wave front, which is generally solved by the finite element method. The numerical treatment of this complex system as part of a virtual heart model is challenging due to the necessity of fine spatial and temporal resolution of the domain. Therefore, efficient surrogate models are needed to predict the electrical activity in the heart under varying parameters and inputs much faster than the finely resolved models. In this work, we discuss an adaptive, projection-based reduced-order surrogate model for cardiac electrophysiology. We introduce an a posteriori error estimator that can accurately and efficiently quantify the accuracy of the surrogate model. Using the error estimator, we systematically update our surrogate model through a greedy search of the parameter space. Furthermore, using the error estimator, the parameter search space is dynamically updated such that the most relevant samples get chosen at every iteration. The proposed adaptive surrogate model is tested on three benchmark models to illustrate its efficiency, accuracy, and ability of generalization.