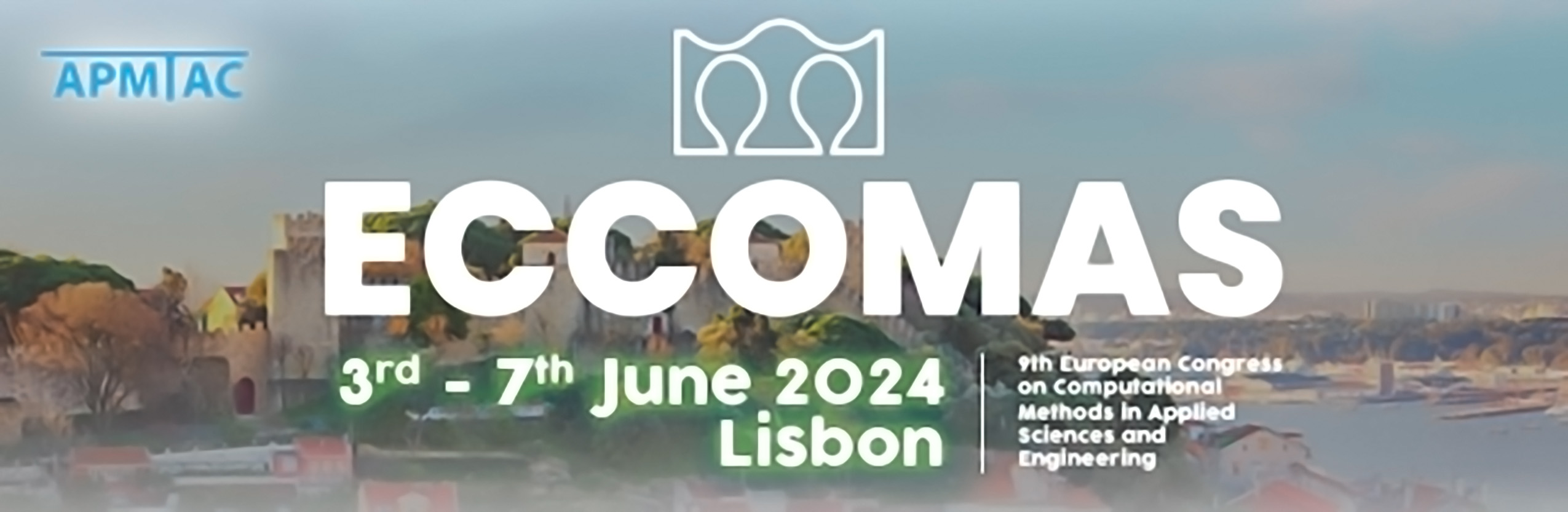
Fast Solution of Partial Differential Equations with Neural Green's Operators
Please login to view abstract download link
A wide range of physical systems is described in terms of first-principle partial differential equations (PDEs). However, for realistic, multi-scale systems, numerically solving these PDEs is typically computationally expensive. Reduced order models can offer computationally cheaper alternatives, however, depending on the application, these may lack sufficient descriptive detail of the relevant physics. This project aims at bridging this gap by setting up a universal framework for fast numerical solution of PDE systems, by using neural network architectures to approximate the PDE’s Green’s function. This ‘neural Green’s operator’ (NGO) acts as a fast surrogate of the PDE’s solution operator: it maps the PDE’s input functions (forcings, boundary conditions, material parameters) to its solution. Additionally, a trained NGO can act as accelerator of existing numerical codes, by providing suitable preconditioners and first guesses. The final goal of this project is the application of NGOs to first-principle nuclear fusion plasma models, which may open doors to more efficient fusion reactor optimization and real-time plasma control. However, we started with benchmarking the method on simpler canonical PDE problems, on which we will present an overview.