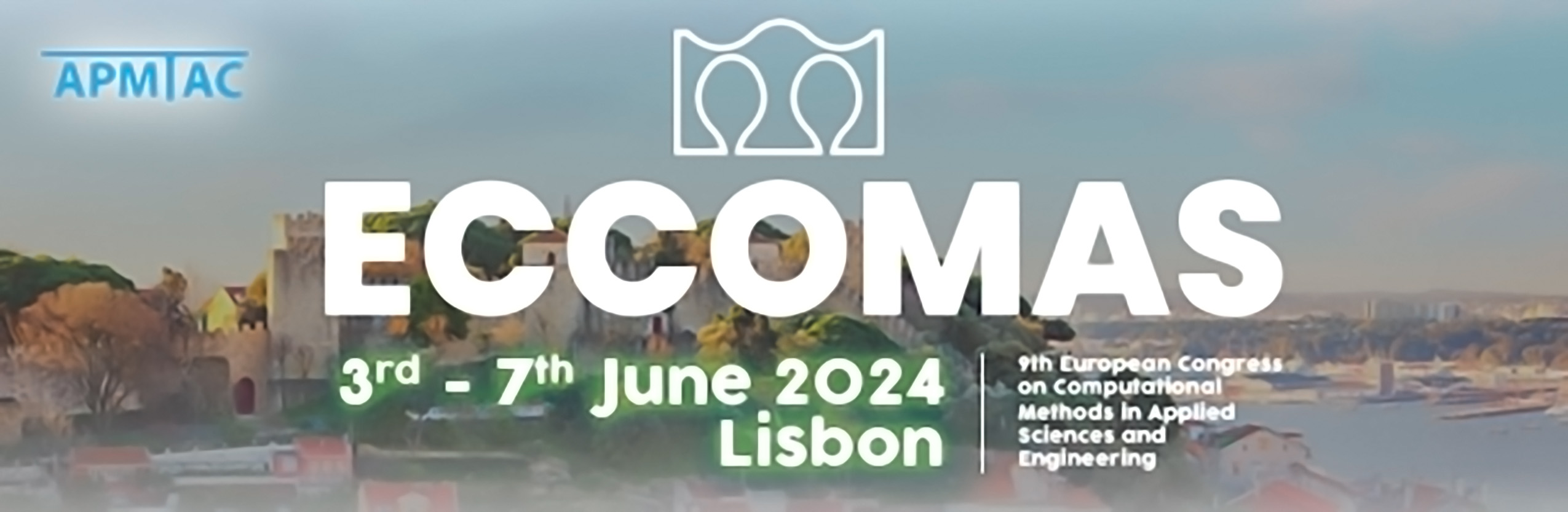
Meta Multifidelity Estimators for Uncertainty Quantification within Outer-Loop Applications
Please login to view abstract download link
For uncertainty propagation within outer-loop applications, such as reliability-based design optimization, we introduce new meta multifidelity estimators which simultaneously leverage multiple sources of variance reduction, including multifidelity Monte Carlo, importance sampling, and information reuse. Our new meta estimators yield a pseudo-multiplicative amount of variance reduction, and we derive asymptotic formulae for the variance reduction of our meta estimators in the limit where the number of outer-loop iterations is large. Using data-driven surrogates and biasing densities, we demonstrate our meta estimators on an uncertainty quantification problem occurring within a design optimization loop from a nuclear fusion application, namely energetic particle confinement during stellarator coil design optimization. For realistic optimization trajectories, we find our measured speedups agree with theoretical and asymptotic predictions, and moreover each meta estimator outperforms its constituent variance reduction techniques. We observe that the highest performing meta estimator, which leverages all three variance reduction sources, yields two orders of magnitude speedup compared to standard Monte Carlo estimation at the same computational budget.