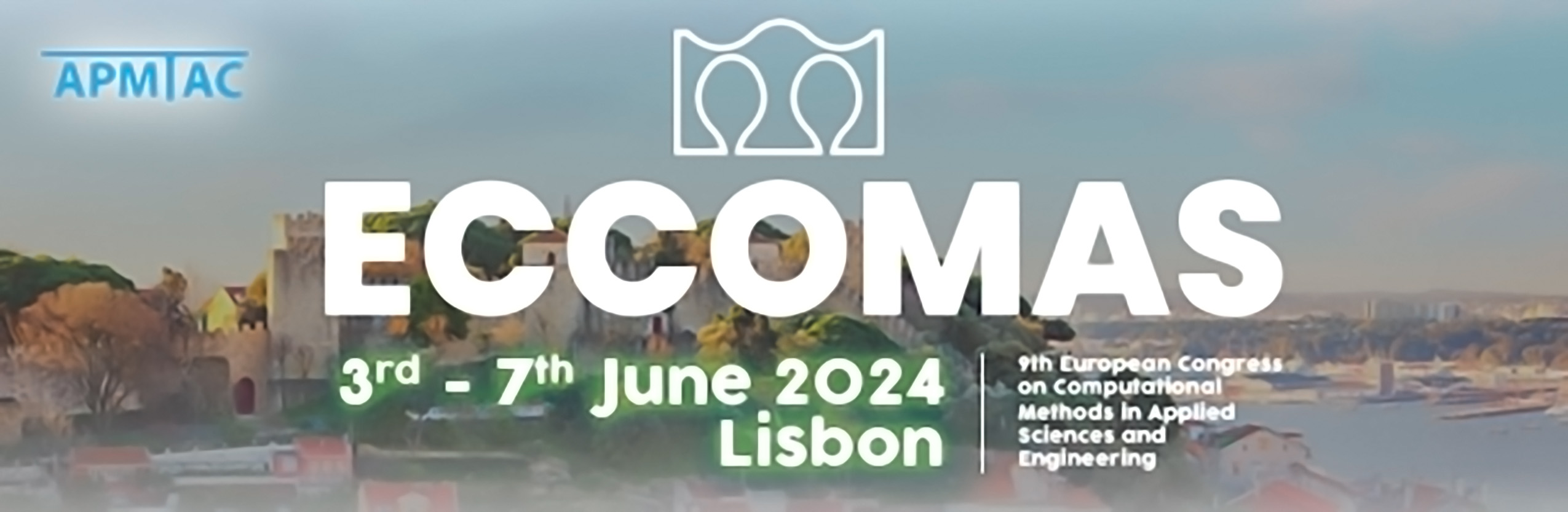
Improving Accuracy in Shape Generation of Motors Using Generative Models
Please login to view abstract download link
In the conventional geometry design of IPM motors for automobiles, designers have to manually design the geometry and then repeatedly evaluate the performance of the motor using finite element analysis (FEA) to see if it meets the required performance. To obtain the desired designs, the designers have to conduct trial and error explorations. However, such exploratory trials are highly dependent on the knowledge and skills of the designer. It requires a large amount of time for repetitive calculations. In recent years, with the improvement of computational performance, design methods using deep generative models have been studied. Unlike optimization, deep generative models have the advantage in generating a wide variety of shapes in a short time. In this study, an IPM motor shapes are generated using generative adversarial networks (GAN), and the accuracy is improved by a physics-guided GAN model. A GAN model, i.e. conditional variational autoencoder / Wasserstein generative adversarial network with gradient penalty (cVAE/WGAN-gp), to generate shapes that satisfy the performance requirements, i.e. torque and area. To improve accuracy, the model was re-trained with a physics-based model that incorporates the results of the FEM analysis into the model. In the conventional physics-based model, the general-purpose software cannot be directly incorporated into the model because the back propagation cannot be performed. However the physics guided GAN can use general-purpose software to train GAN models. The PG-GAN is utilized in the motor generation task, and the model generated shapes with performance values closer to the required performance than the conventional method.