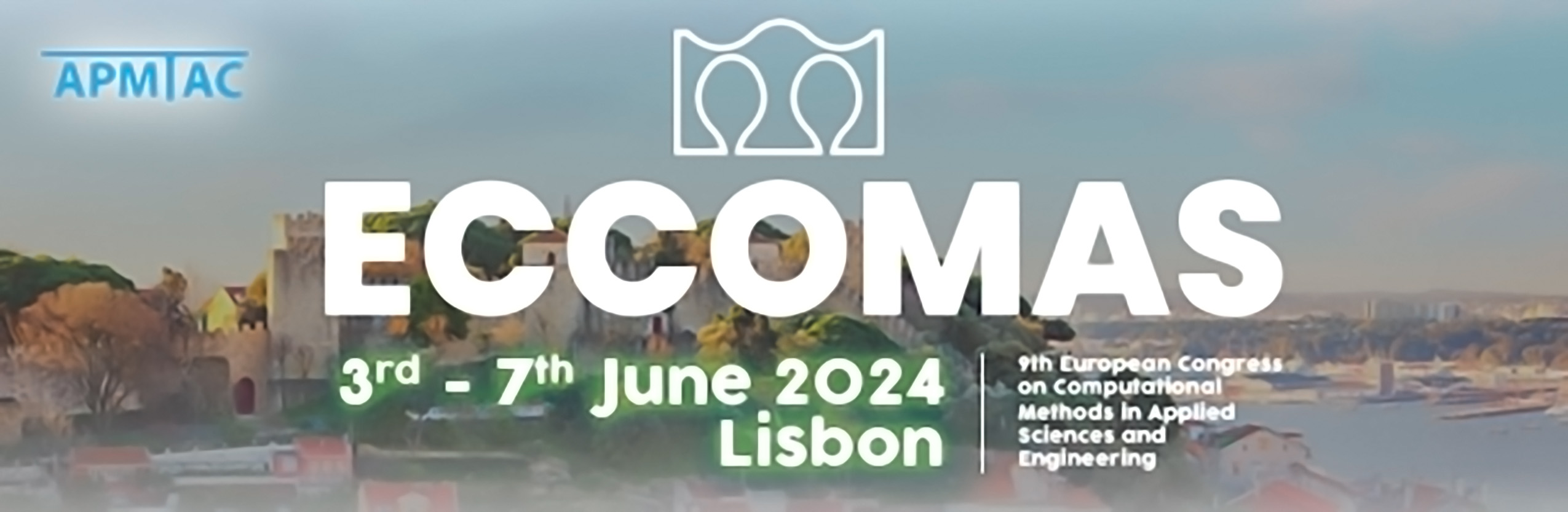
Enhancing Tall Timber Building Design through Multi-Building Bayesian Inversion
Please login to view abstract download link
Bayesian inference has emerged as a powerful statistical tool for deducing design parameters in simulation models, facilitating predictions of displacements, deformations, and dynamic characteristics of building structures. This methodology utilises measurements of structural behaviour, enabling the refinement of finite element (FE) models, commonly used for tall timber buildings. The application of Bayesian inversion in this context may serve two objectives: firstly, the creation of a digital twin that dynamically updates the FE model based on real-time building behaviour, aiding in tracking structural ageing for critical infrastructures like bridges, hospitals, and nuclear power plants. Secondly, it may allow us to comprehend the structural response of unconventional structures, contributing to the enhancement of their design procedures. One challenge inherent in the Bayesian framework is the persistence of systematic errors within the model, stemming from simplifications made during the translation of real-world scenarios into numerical simulations. Even with the integration of stochastic elements to represent uncertainties, the updating process tends to introduce a systematic error that seeks to reconcile unknown factors. Though the updated will probably results in an improved fit to the observed building behaviour but may compromise the original physics-based parameters, subsequently impacting the generalisation capability of the updating process. This contribution proposes a novel approach to address this challenge by focusing on the generalisation capability of Bayesian inversion through the analysis of multiple buildings. Rather than relying solely on the measurements from a single structure, our methodology considers data from several buildings. By leveraging a multi-building dataset, we aim to enhance the robustness of the Bayesian inversion, thereby improving its ability to generalise across diverse tall timber building scenarios. Through this innovative approach, we seek to refine the design procedures for tall timber buildings, ensuring a more accurate representation of their dynamic behaviour while preserving the integrity of the underlying physics-based parameters.