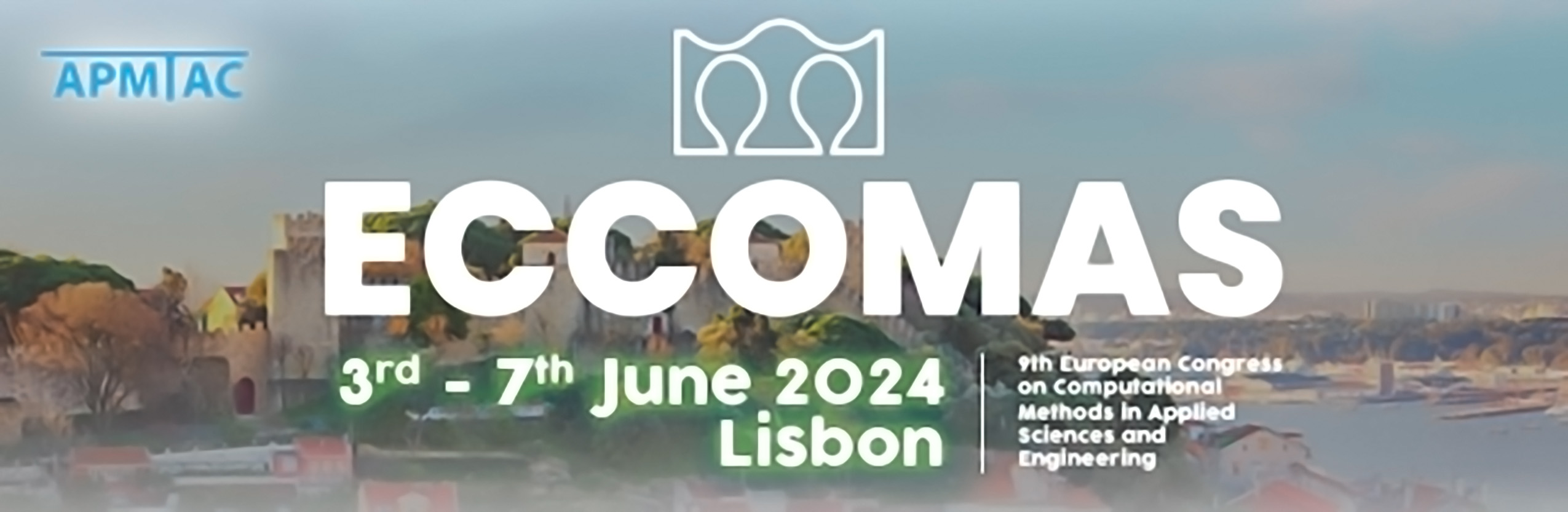
Physics-Informed Wavelet Neural Operator for Data-free Learning of Parametric Partial Differential Equations
Please login to view abstract download link
Partial differential equations (PDEs) are widely used for modeling complex constitutive laws of physical systems across various corners of science and engineering disciplines. During the design and analysis of physical systems, these PDEs are repeatedly solved for various input conditions, e.g., different initial and boundary conditions, input parameters, and forcing functions. To solve these so-called parametric PDEs, laborious numerical computational tools such as finite element methods and finite volume methods are adopted in a Monte Carlo framework, which dramatically increases the computational cost. With massive improvements in representation learning, deep neural operators have proven to be an effective and efficient scientific machine learning tool for learning solutions of these complex parametric PDEs. By projecting the input functions into a common subspace, the deep neural operators learn the solution operator of a family of PDEs. Thereby, once trained, a single neural operator can predict solutions of PDEs for varying inputs without repeated independent runs from scratch. A recently proposed Wavelet Neural Operator (WNO) is one such neural operator that harnesses the time–frequency localization property of wavelets to effectively learn useful features in the PDE domain [1]. However, successful training of WNO requires thousands of labeled training data. Relying completely on costly conventional solvers for labeling data and subsequently training WNO with generated labeled data leads to a time-consuming process. Motivated by the data bottleneck, we introduce the physics-informed WNO using a stochastic-projection-based gradient estimation scheme for learning the solution operators of parametric PDEs without labeled training data, thereby achieving 100\% data efficiency. We validate the efficacy of the proposed PIWNO on nonlinear spatiotemporal systems like Burgers, Nagumo, and Allen-Cahn equation. With a mean squared error of approximately 0.03\%--2.5\%, we achieve a computational speedup of around 300\%--800\% over conventional solvers. REFERENCES [1] T. Tripura and S. Chakraborty. Wavelet neural operator for solving parametric partial differential equations in computational mechanics problems. Computer Methods in Applied Mechanics and Engineering, 404, 115783, 2023.