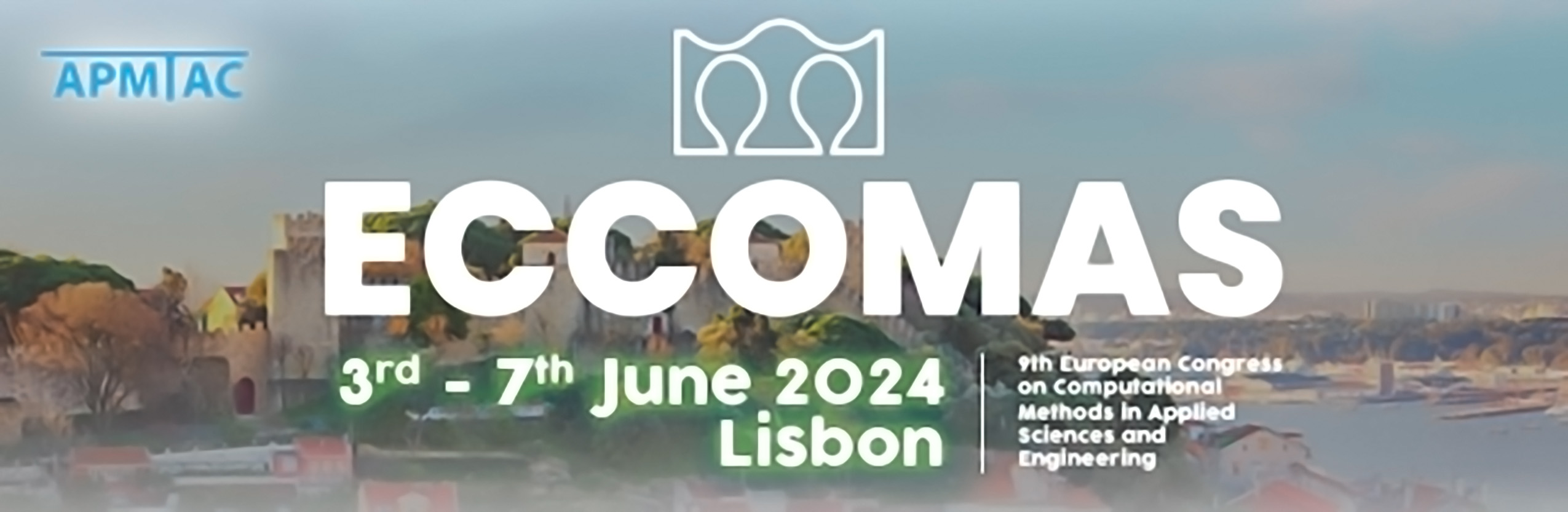
A Multi-fidelity Strategy for Optimization under Uncertainty with Robust Constraints
Please login to view abstract download link
Effective solutions for optimizing systems under uncertainty are crucial for ensuring the success of missions, enhancing the reliability of robotics, and preventing system failures. However, the conventional optimization under uncertainty approach often comes with substantial computational costs, making real-time implementation or thorough uncertainty propagation challenging using a costly simulation model alone. In this context, we propose an innovative multi-fidelity optimization under uncertainty algorithm with robust constraints that leverages inexpensive, low-fidelity simulations to alleviate computational burdens. We employ a sample average approximation technique to transform the traditionally stochastic optimization problem into a deterministic framework. Our strategy involves integrating high-fidelity samples with a more extensive set of cost-effective low-fidelity samples for precise estimation of both the objective function and constraints. Specifically focusing on general convex optimization problems, we study the impact of multi-fidelity constraints on the robust optimization results. The incorporation of low-fidelity models may introduce bias into the constraint estimate, impacting optimization results while concurrently aiming to diminish the variance of the robust constraint estimate. This study conducts a comprehensive analysis of the trade-offs associated with multi-fidelity optimization, providing insights into how errors in low-fidelity sampling propagate through the optimization problem. Additionally, we present preliminary results on a trajectory optimization under uncertainty problem, demonstrating safe and robust trajectory generation. We test a simple bi-fidelity strategy for accelerating trajectory optimization under uncertainty in the presence of robust constraints. Specifically, we accelerate the optimization using additional samples of low-fidelity models for assessing whether or not a risk constraint is violated. The low-fidelity model's purpose is thus to reduce the uncertainty in the estimate of constraint violation, at the expense of introduction of bias. Our empirical test on a simulation of glider dynamics tasked with avoiding a region indicates an order-of-magnitude less failures using the bi-fidelity strategy.