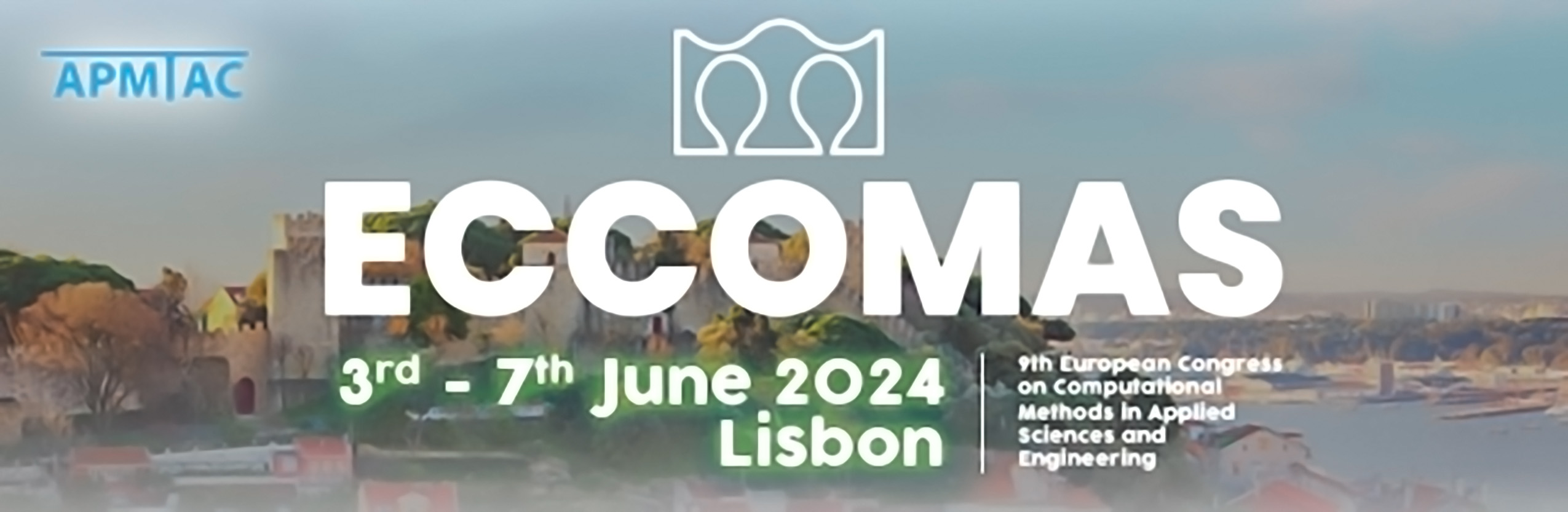
Integrating Cell Cycle Data from In Vitro and In Silico Models to Target Radiation Response and Cancer Resistance After Radiation Exposure
Please login to view abstract download link
Oncology is marked by the relentless pursuit of effective treatments, with radiation therapy (RT) being a cornerstone for many types of cancer. However, the efficacy of this approach is often limited by radiation-resistance, particularly in colorectal cancer (CRC), the third most common and second most lethal cancer worldwide. This study provides new insights on the mechanisms of radio-resistance by comparing cellular responses in different cell lines, using both experimental in vitro models and a computational approach. We initially focused our research on the effects of ionising radiation (IR) on cell cycle regulation on two human CRC cell lines, Caco-2 and DLD-1. We employed flow-cytometry to quantify the alterations in cell cycle dynamics post X-ray exposure up to 10 Gy, revealing a dose-dependent elongation in G2-phase and G1-phase later accumulation. These findings highlight the cellular strategies to cope with DNA damage induced by IR. To translate these observations into a predictive framework, we developed a deterministic compartmental model based on Ordinary Differential Equations (ODEs) that simulates cell cycle transitions, tumour growth, and cell death rates, offering a population-level view of how surviving cells redistribute post-irradiation. The output parameters of our model hold biological significance and provide quantitative measures of cell radio-resistance, crucial for the early response period up to 72 hours post-exposure. Parameters were calibrated against in vitro data, including cell cycle phase distributions and independent measures such as cell doubling time and DNA synthesis duration. A previous version of the model was adjusted to reproduce the dynamics of irradiated normal cells as a proof of concept. Starting from the perturbed cell cycle profile, our model provides a predictive framework to interpret the cellular perturbations triggered by radiation as a pre-clinical tool and could offer insights into the optimization of RT dosing and scheduling. The robustness of our model is further validated by its application to other cell line datasets available in the literature, such as ovarian cancer, with different levels of radio-sensitivity. Concluding, by forecasting the growth curves of irradiated cancer cells, the model can expose the complexities of cancer cell radio-resistance, integrating in vitro data with computational modelling and comparing different cancer types, with the aim to enhance the effectiveness of RT.