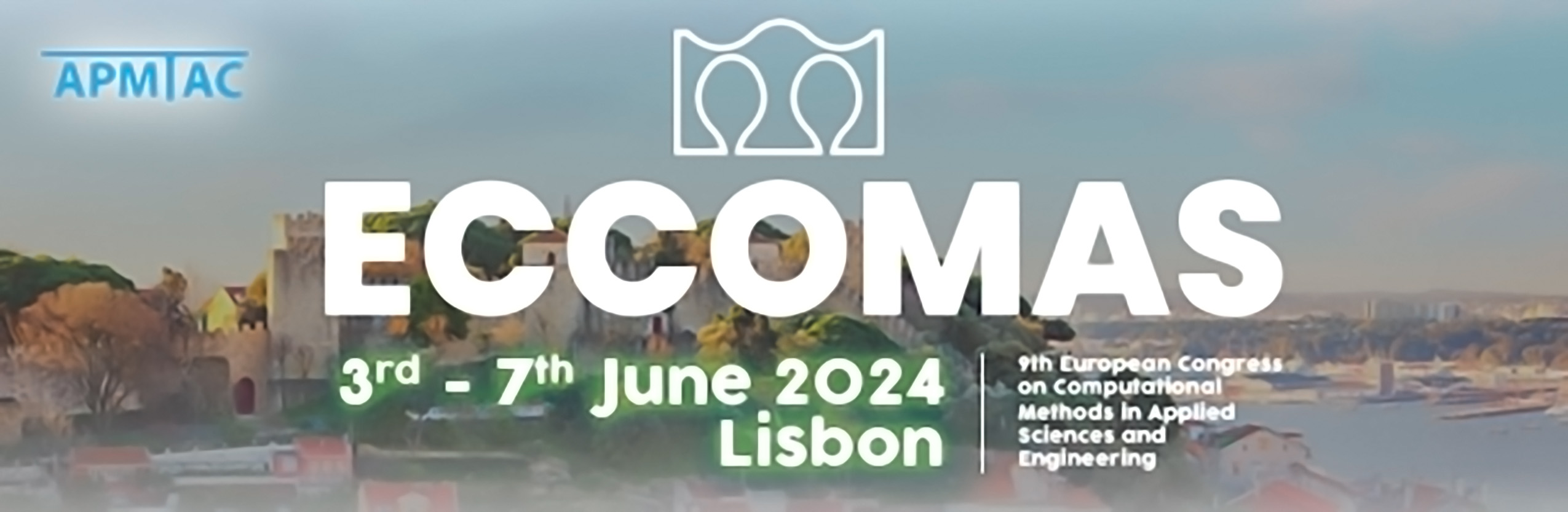
A data-driven physics-based model for predicting prostate cancer progression from the PSA blood test
Please login to view abstract download link
Prostate cancer ranks as the second most common cancer in men worldwide, as reported by the World Health Organization (WHO). The current diagnostic approach, which measures the prostate-specific antigen (PSA) biomarker in blood, often fails to distinguish between stable cases and those progressing to rapidly fatal tumors [1]. Despite notable advancements in simulating prostate cancer [2], a critical gap exists, necessitating the development of a model that precisely accounts for patient-specific growth dynamics. Here, we propose a data-driven physics-based model to predict tumor growth based on patient’s PSA blood test results. To achieve that, we generate a three-dimensional prostate representation from patient’s T2-weighted magnetic resonance image (MRI) sequences, incorporating cellular-level details such as cellularity, vascularization, and tumor location. We then apply a novel mathematical model based on partial differential equations (PDEs) to simulate the evolution of the PSA levels and tumor growth. Our model considers both tumor-produced PSA and its vascular transfer to the bloodstream, hence linking serum PSA dynamics to tumor progression [3]. We also incorporate a deep learning algorithm to mirror patient-specific serum PSA levels more accurately, employing a neural network within the PDE framework to estimate the proportion of proliferating tumor cells using simulation and patient data. This methodology provides a dynamic representation of tumor evolution based on PSA levels. Validation with real patient data demonstrates our methodology’s potential in forecasting prostate tumor evolution based on patient follow-up serum PSA levels.