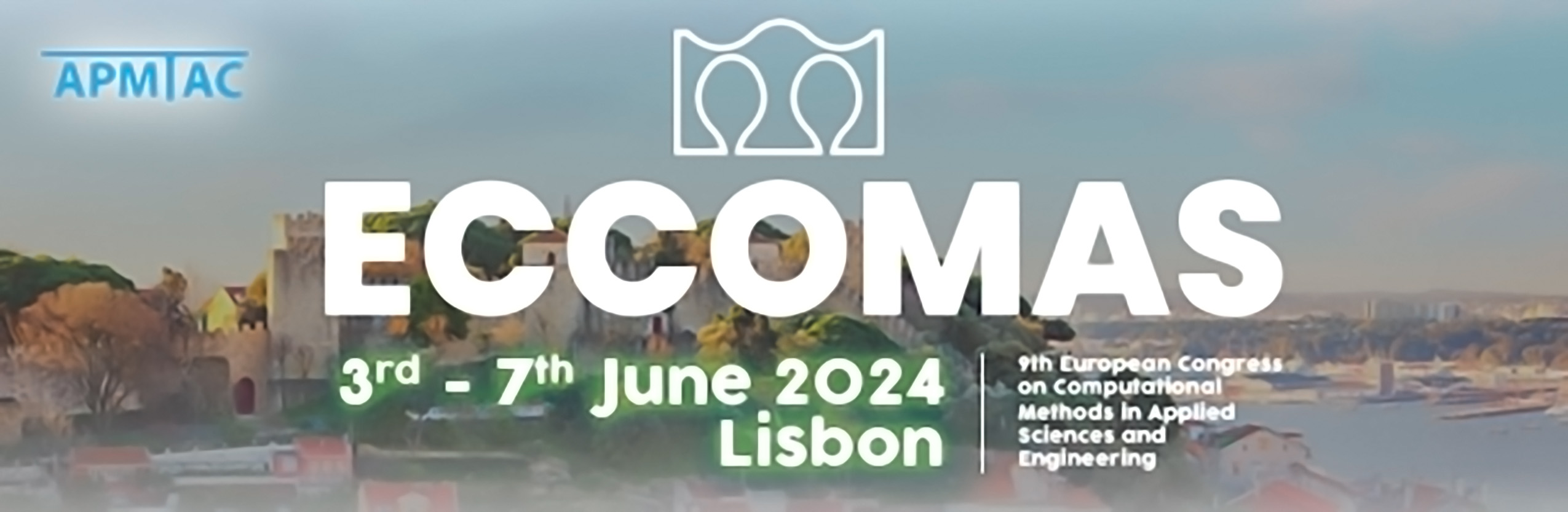
An adaptive Approach for Surrogate Modeling Based on Full Maximum a Posteriori Estimation of Model Error for Efficient Calibration of Numerical Codes
Please login to view abstract download link
Computer codes are crucial for solving engineering problems. However, these codes are based on complex models involving several context-specific parameters that may be unknown. To infer the best values of these parameters, model predictions are compared to experimental observations. Besides, these models cannot accurately reproduce real-world processes. The difference between model predictions and reality is due to the model error, and it should be accounted for in the identification of the model parameters~\cite{koh_paper}. In this work, we rely on a Bayesian calibration technique called the Full maximum a posteriori method (FMP)~\cite{papier_1}, where a statistical model error is identified for every possible model parameter value. As for many other Bayesian methods, one limitation of FMP is the large number of model solutions that need to be computed and the associated numerical cost. Replacing the numerical code with a surrogate model is one possible solution. We propose an adaptive approach for constructing a surrogate model of the computer code that minimizes the approximation error on regions of the parameter domain with high FMP posterior value. Specifically, we build a first surrogate using simulations at a few parameter values and solve the FMP calibration problem. The initial design of experiments is enriched sequentially by drawing new parameter values from the FMP posterior distribution before updating the surrogate model. As a surrogate, we use the Gaussian process model. This approach is applied to a complex two-phase flow model to calibrate the parameters of the coalescence and breakup processes in the IATE equations in the bubbly flow regime. Results show that the proposed method improves computational efficiency by reducing the number of actual model evaluations required for surrogate construction compared to conventional space filling designs. Additionally, the surrogate we obtain is a reliable approximation of the computer model.