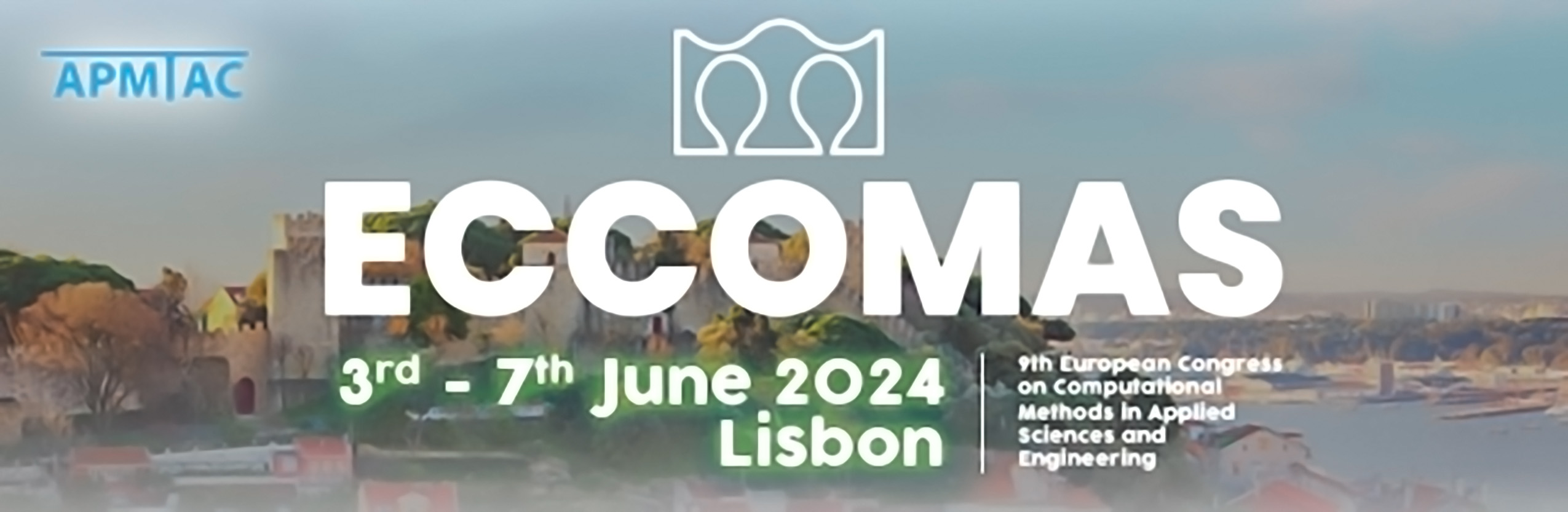
Multi-fidelity Machine Learning for Quantifying Uncertainty in the Progressive Damage of Composites
Please login to view abstract download link
High-fidelity surrogate model construction is computationally demanding to investigate problems such as damage mechanisms of composite laminates. A multi-fidelity (MF) surrogate model coupled with the Gaussian process is constructed to characterise the impact of uncertainty on the prediction of progressive damage of composite laminates [1, 2]. The coarse mesh FE model is taken as a low-fidelity model and the fine mesh FE model as a high-fidelity model. A smaller amount of high-fidelity model data with more low-fidelity data is incorporated into multi-fidelity surrogate model construction. In this work, we have propagated the inherent randomness at the source level and quantified its effect on the progressive damage of composite laminates [3]. A multi-fidelity surrogate-based probabilistic study is carried out to investigate the effect of source uncertainty of composites on the global response. It is shown that the computational cost of performing uncertainty quantification is reduced by using MF-based surrogates as compared to high-fidelity surrogates.