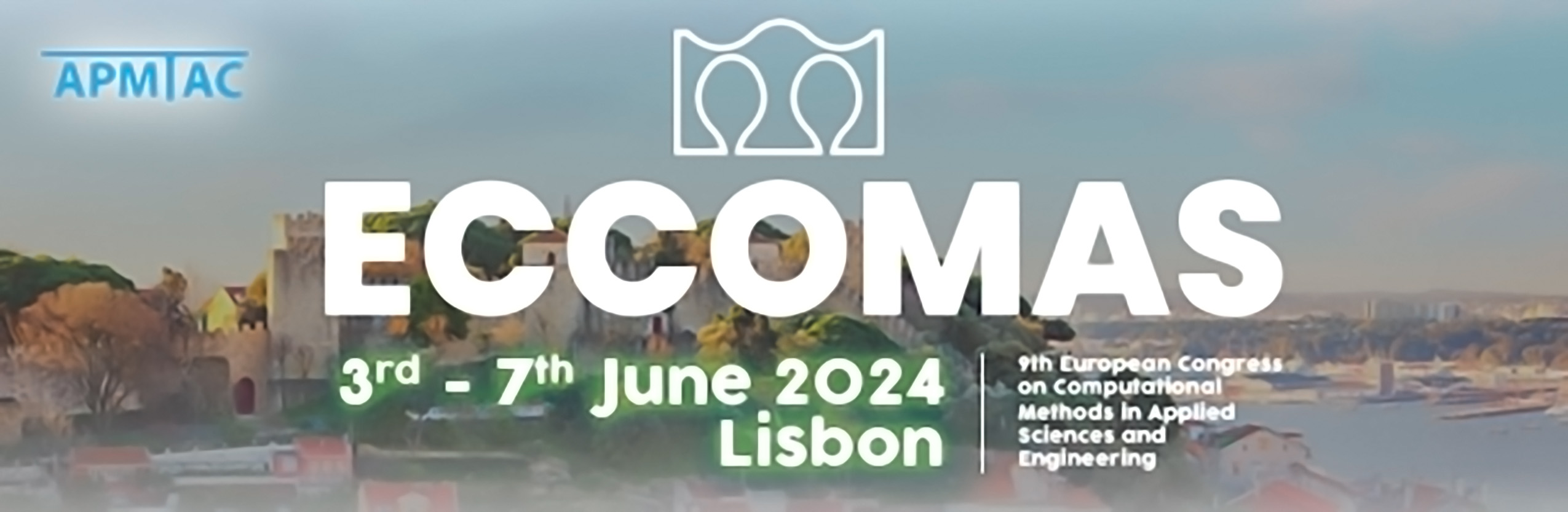
Solution Space Parametrization for Immiscible Compositional Problems using Neural Networks
Please login to view abstract download link
Recently deep learning approaches to modelling of flow through porous media have shown that machine learning algorithms can function well as a surrogate for reservoir simulation. These algorithms achieve orders of magnitude faster runtimes than numerical simulations, while maintaining sufficient accuracy, and are further applicable to a wide range of ensemble-based techniques. In this work we train a deep neural network to predict the transport solution for a multi-component compositional simulation with observations from a solution of a discretised nonlinear model. Training is carried out with labelled as well as unlabelled collocation points sampled uniformly and randomly from the entire solution space. The discretised form of the residual equation is included in the loss function and provides an additional training signal to both expedite training and minimize the reliance on labelled data when working in a higher dimensional parameter space. The neural network is utilised within a cascading solver and effectively parametrizes the solution in control volume with respect to its current state and the state of the adjacent grid block at the updated time step. In addition, the neural network can be used for solution methods where the transport and flow solution are effectively decoupled (e.g. sequential implicit solver) and is furthermore applicable as a preconditioner to shorten solving time.