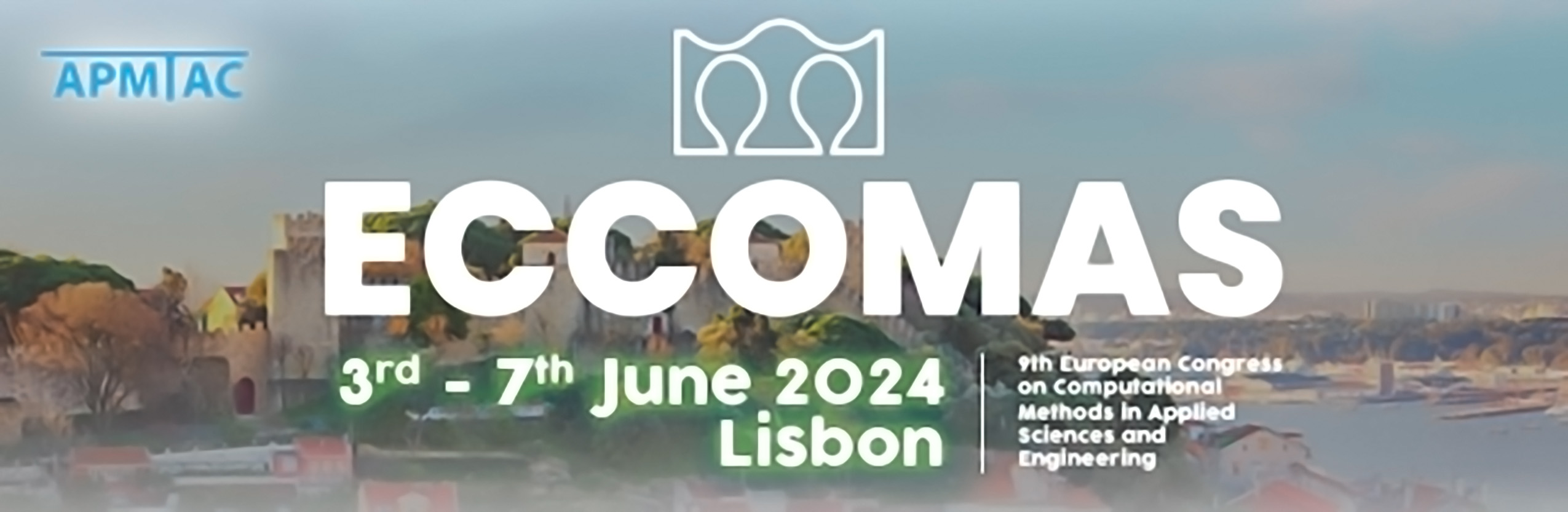
Leveraging Conservation Form of Hyperbolic PDEs to Enhance the Solution Learning by PINNs for Phenomena with Moving Shocks.
Please login to view abstract download link
Conservation phenomena and associated differential equations are ubiquitous in Nature and a lot of research investment goes into simulation of these systems. A huge class of such partial differential equations (PDEs) can be categorized into Hyperbolic PDEs [1]. A challenging feature of such PDEs is that there can be possibility of shock devel- opment. Even a smooth initial data can evolve into discontinuous data at later times. Detection and handling of such shocks is a key challenge in simulation of such systems [1]. Physics-inspired neural nets (PINNs) based methods also face these challenges in simulating phenomenon with shocks. Diagnosing and alleviating various issues in using neural networks for such PDEs, is a vibrant research field. Many works [2, 3, 4] point out different shortcomings of simple PINNs and propose modifications like adding extra loss terms, adaptive sampling, specialized training procedures, etc. In our work, we study the possibility of using PINNs that are based on conservation forms of the PDEs rather than the differential form, for simulation of systems with moving shocks. Unlike the traditional PINNs that use neural nets as an ansatz for the solution, we use neural nets to model the integrals of the solution instead of the solution itself. Then leveraging the fundamental theorem of calculus, we can train them using Physics-informed loss. We study these modified PINNs in different types of linear and nonlinear 1-d cases and show that they are much better at capturing the shock locations than the “vanilla” PINNs and give overall better solutions. In case of development of a discontinuity, when the differential form breaks down, these network tend to give correct solution without adding viscosity which is a must for vanilla PINNs. In case of more than one moving shocks, our integral-based formalism gives significantly better results than vanilla PINNs.