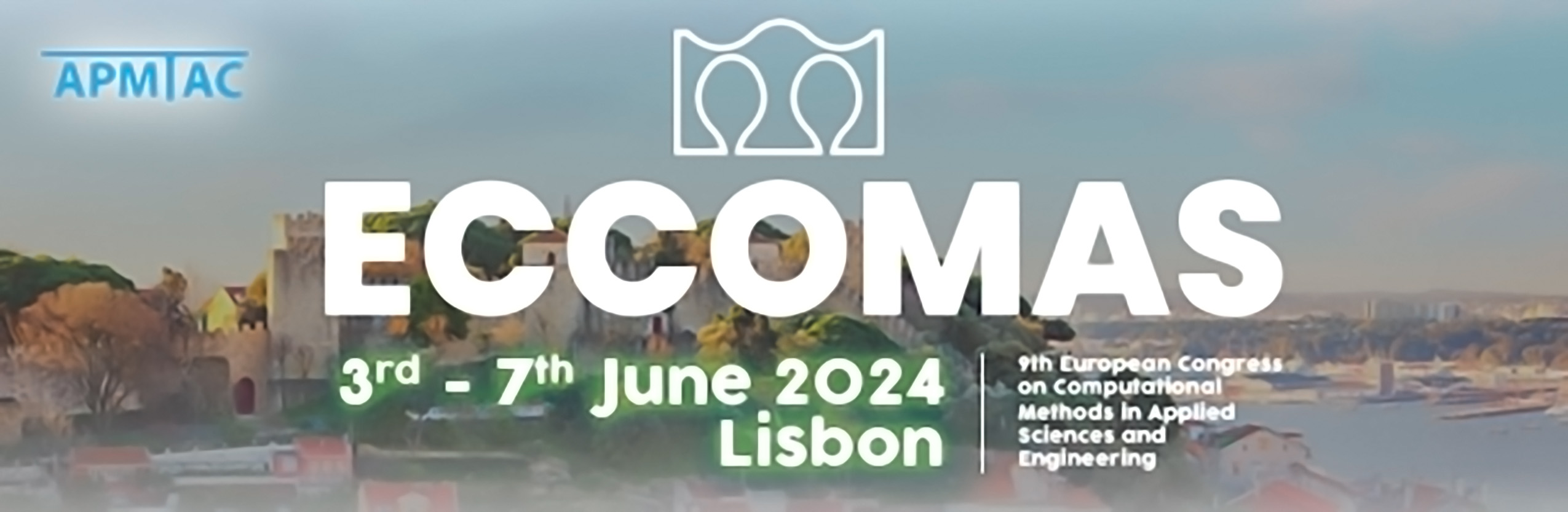
Enhancing Data Assimilation and Uncertainty Analysis in CCUS Projects Through AI Integration
Please login to view abstract download link
This study focuses on combining machine learning (ML) and data assimilation (DA) methods, a rapidly growing area, with a particular focus on surrogate models for Carbon Capture, Utilization, and Storage (CCUS) projects, ensuring the retention of high-fidelity physical results in posterior states. We evaluate the surrogate modeling capabilities of two distinct ML models, Fourier Neural Operators (FNOs) and Transformer UNet (T-UNet), in the context of CO2 injection simulations within channelized reservoirs. We introduce the Surrogate-based Hybrid Ensemble Smoother with Multiple Data Assimilation (SH-ESMDA), a novel method that integrates pressure observations from four distinct monitoring points within the reservoir. This integration is key to accurately quantifying the uncertainty of both CO2 molar fraction distribution and reservoir pressure. By utilizing data from these strategic locations, SH-ESMDA aims to significantly enhance the precision of our assessments. This method applies FNOs and T-UNet as surrogate models, potentially accelerating the standard ESMDA process by at least 50%, dependent on the number of assimilation steps. Additionally, we propose the Surrogate-based Hybrid Randomized Maximum Likelihood (SH-RML), a variational data assimilation approach that utilizes the randomized maximum likelihood (RML). Here, both FNO and T-UNet provide the gradient computation for optimizing the objective function, while a high-fidelity model is employed for computing posterior states. Comparative analyses demonstrate that the SH-RML method yields more accurate results in uncertainty quantification over the ESMDA for the case study. This is reflected in smaller errors in fitting observational data and estimations of reservoir permeability that align more closely with the geological characteristics of the channelized reservoir under study.