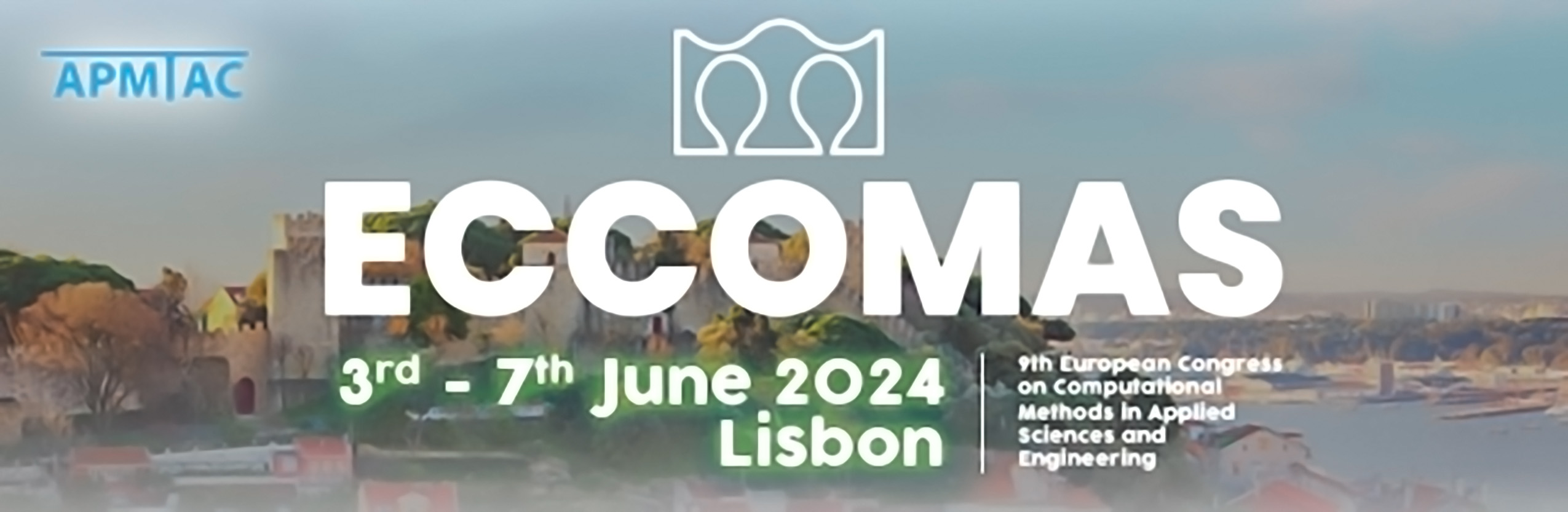
N-Adaptive Ritz Method: A Neural Network Enhanced Computational Mechanics Framework
Please login to view abstract download link
Conventional finite element methods are known to be tedious in adaptive refinements due to their conformal regularity requirements. Meshfree methods, such as the Reproducing Kernel Particle Method, relaxed the discretization and approximation regularity requirements in finite elements, however the enrichment functions for adaptive refinements are not readily available in general applications. The fast-growing research and development in data science, machine learning and artificial intelligence offer new opportunities for the development of new paradigms in scientific computing. This talk presents a neural network (NN) enhancement of Galerkin solution for weak and strong discontinuities, as well as for solution enhancement with a fixed discretization, called N-adaptivity. The flexibility and adaptivity of the NN function space are utilized to capture complex solution patterns that the conventional Galerkin methods fail to capture. The NN enrichment is constructed by combining pre-trained NN blocks with an additional untrained NN block. The pre-trained NN blocks learn specific complex solution patterns during the offline stage, enabling efficient enrichment of the approximation space during the online stage through the Ritz-type energy minimization. The NN enrichment is introduced under the Partition of Unity (PU) framework, ensuring convergence of the proposed method. REFERENCES [1] J. Baek, J. S. Chen, K. Susuki. A neural network‑enhanced reproducing kernel particle method for modeling strain localization, International Journal for Numerical Methods in Engineering, 123(18): 4422–4454, 2022. [2] J. Baek and J. S. Chen, A neural network-based enrichment of reproducing kernel approximation for modeling brittle fracture, Computer Methods in Applied Mechanics and Engineering, 419: 116590, 2024.