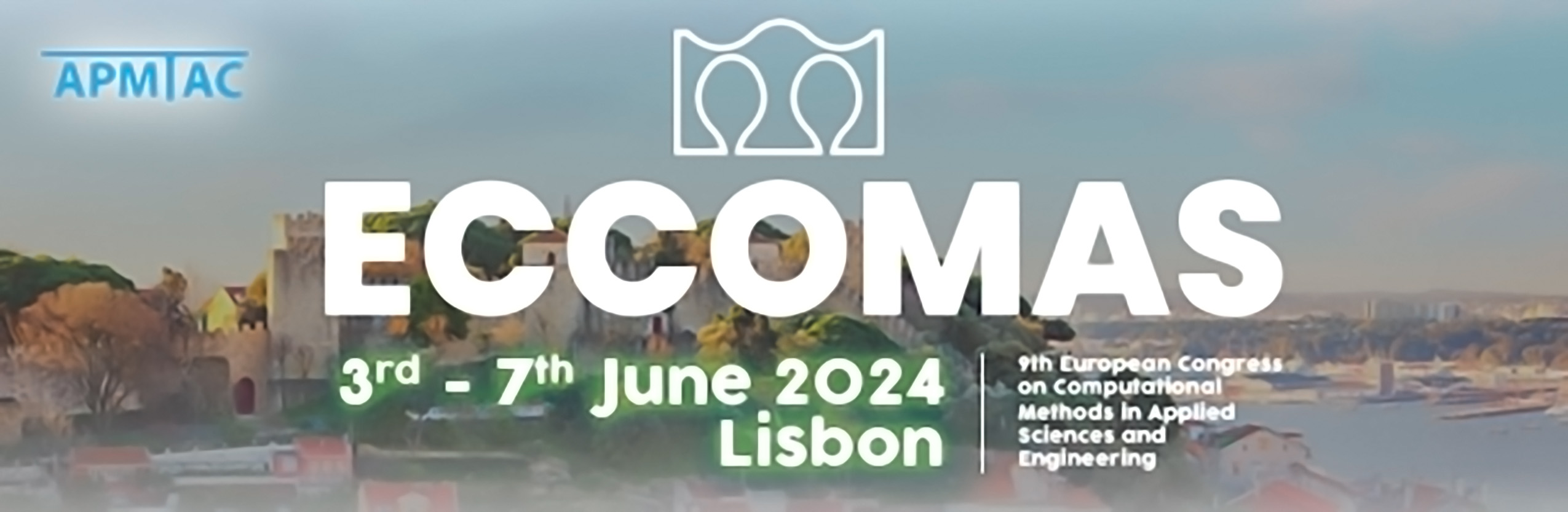
Physics informed Graph Neural Networks – towards real-time applications for haptic devices
Please login to view abstract download link
and solved using the Finite Element Method (FEM), Graph Neural Networks (GNN) can successfully predict the temporal evolution of nonlinear complex phenomena [3]. Often accused of being ‘black box’ solutions to complex problems, neural networks can be thermodynamics-aware [1], which means that physical principles can be embedded into the model, thus providing a bias to physically consistent solutions. In this context the use of the GENERIC [2] formalism provides an elegant and efficient way to integrate the principles of thermodynamics into the integrator used to solve the time evolution problem. In this work, Thermodynamics-Informed Graph Neural Networks (TIGNN) are used to estimate the deformation of thin shells, subjected to a point deformation. Nonlinear phenomena such as the snap-though are successfully captured by the model. Moreover, the inference results obtained from the trained model are tailored to feed a haptic device (haptic glove + augmented reality system) able to provide a quasi-real-time experience to the user. In future this solution is intended to evolve into a robust tool for industrial usage. The aim is to provide real time haptic information on the manipulation of thin shell structures to factory workers and assembly line engineers, thus allowing for realistic assembly line operator training and manufacturing process optimization. REFERENCES [1] Q. Hernandez, A. Badías, D. González, F. Chinesta and E. Cueto. Deep learning of thermodynamics-aware reduced-order models from data. Computer Methods in Applied Mechanics and Engineering, 379, 113763. 2021. [2] H. C. Öttinger, Beyond equilibrium thermodynamic, John Wiley & Sons, Inc., 2005. [3] Q. Hernandez, A. Badias, F. Chinesta and E. Cueto. Thermodynamics-informed Graph Neural Networks, IEEE Transactions on Artificial Intelligence, 2022.