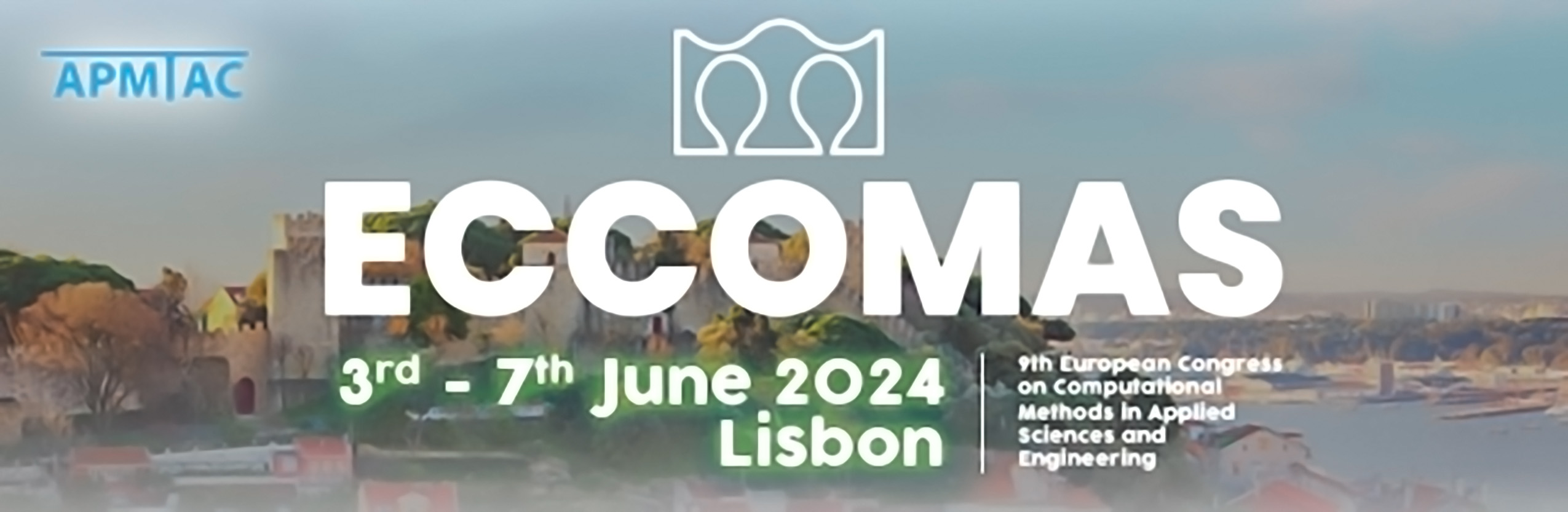
Multi-Fidelity Surrogate Model for Efficient Aerodynamic Predictions
Please login to view abstract download link
Multi-fidelity and surrogate modelling represent a promising advance in improving the efficiency and computational accuracy of aerodynamic data prediction. The integration of machine-learning techniques and the consideration of multi-fidelity contribute to efficient design optimization, reduced experimental costs and overall improved predictive accuracy. We propose the development of a multi-fidelity surrogate model for aerodynamic data prediction at higher fidelity than baseline training data. Based on pressure coefficient (Cp) distribution over airfoils, the model workflow starts with dimensionality and fidelity augmentation, which subsequently serves as the foundation for generating a Cp predictor based on the airfoil geometry. The first part of the research consists of combining the Cp values of a four-digit NACA airfoil with two different fidelities: low-fidelity data from a non-viscous panel method and high-fidelity data from a RANS-type CFD database [1]. Multi-fidelity is implemented using the Multi-fidelity Gaussian Process Regression method, combining a full low-fidelity Cp vector with a sparse vector of high-fidelity data [2]. The rationale for excluding the full vector from the CFD simulation is to emulate the conditions of a wind tunnel test with a limited number of pressure taps while using the remaining Cp values in the airfoil to calculate the reconstruction error. On the basis of the augmented database, which improves its fidelity and dimensionality, the second part of the study aims at building a regression model capable of predicting the Cp distribution at higher fidelity, using the airfoil geometry as input. To improve the cost-effectiveness and efficiency of the regression, we propose the combination of manifold learning techniques with neural networks [3]. A manifold learner, acting as an encoder, maps the Cp data onto a low-dimensional space capable of retaining the intrinsic physical features of the system. Based on the low-dimensional data representation, a neural network is trained at a fraction of the cost. Ultimately, our methodology aims to facilitate rapid, cost-effective and reliable aerodynamic analysis, reducing the need for additional simulations or experiments.