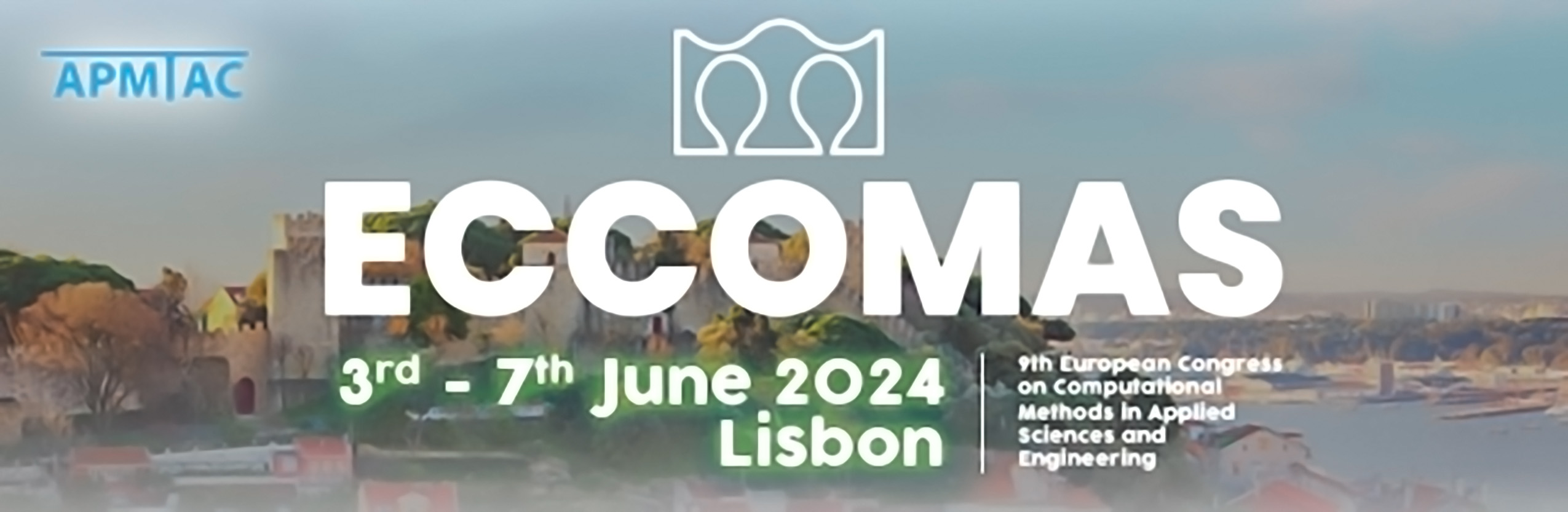
Eulerian MonteCarlo methods for the joint statistics of velocity and scalar fields
Please login to view abstract download link
Turbulent combustion presents challenges in modeling due to the highly non-linear thermochemical processes involved, alongside turbulent heat and mass transfer complexities. Addressing these challenges, a Probability Density Function (PDF) simulation approach proves advantageous by handling local terms without the need of additional models. In PDF methods, turbulent transport aspects remain open, requiring the incorporation of coarse-grained models. Original PDF numerical methods adopted a Lagrangican MonteCarlo (LMC) approach, transporting stochastic particles through computational cells and coupling them with Eulerian variables in Computational Fluid Dynamics (CFD) solvers. Alternatively, Eulerian MonteCarlo (EMC) fields were introduced, leveraging standard numerical methods for Eulerian field integration in CFD codes. EMC fields specifically model the Eulerian flow of probability, using Stochastic Differential Equations (SDE) with time noise, differing from Stochastic Partial Differential Equations (SPDE) with space and time noise. Efforts to improve transport process representation involve joint statistics modeling of velocity and scalar fields, aiming to eliminate the need for gradient closures. However, challenges arise in pressure coupling and turbulent cascade development within stochastic fields due to preserved non-linear transport terms. Application of a joint velocity-scalar EMC method to a highly inhomogeneous case revealed numerical issues mitigated with increased diffusion. Spatial variations finer than computational cell size prompted a reformulation of PDF and SDE equations. A Large Eddy Simulation (LES) spatial filtering technique was employed to address this challenge, enhancing simulation accuracy without the computational expense of a standard LES method. Mesh refinement, though necessary, was less computationally burdensome, focusing on statistically averaged values and their smoother spatial variations. Special attention was given to stochastically representing a close-to-wall boundary layer as a stochastic singular boundary, acknowledging its size relative to computational cells. The resulting numerical method is stable and successfully improves the simulation of a test case, showing its effectiveness in handling the intricacies of turbulent combustion modeling.