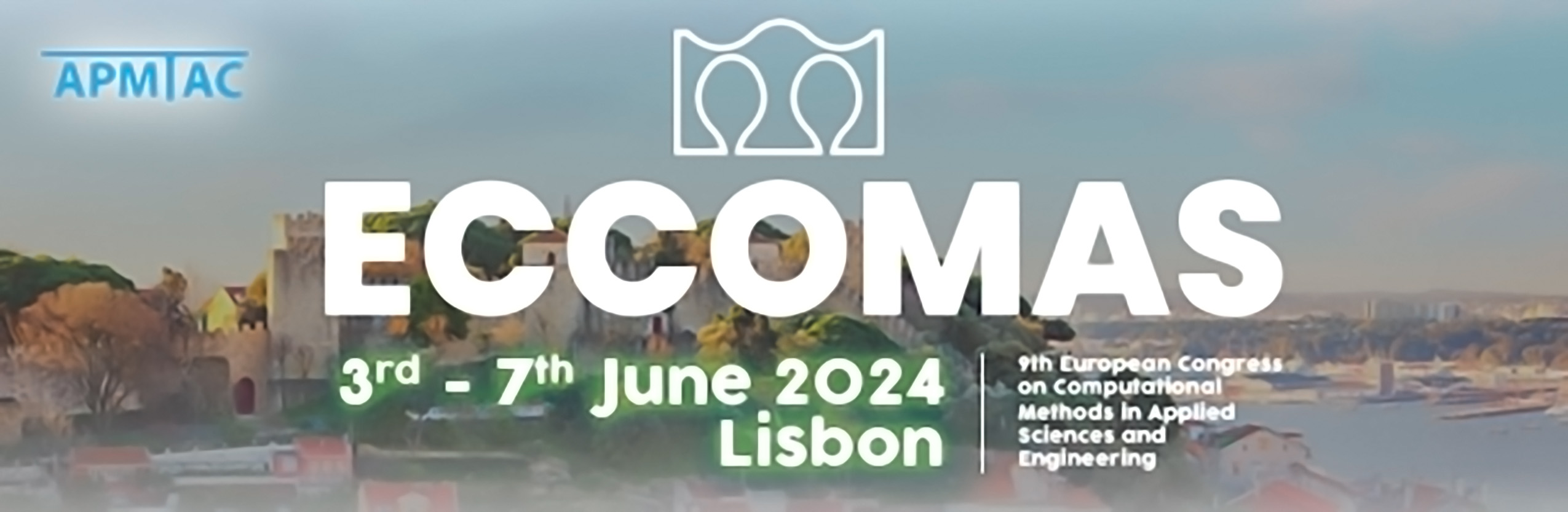
Neural Integration for Constitutive Equations in Small Data
Please login to view abstract download link
Data-driven models based on deep learning algorithms intend to overcome the limitations of traditional constitutive modelling by directly learning from data. However, the need for extensive data that collate the full state of the material is hindered by traditional experimental observations, which typically provide only small data – sparse and partial material state observations. To address this issue, we develop a novel deep learning algorithm referred to as Neural Integration for Constitutive Equations to discover constitutive models at the material point level from scarce and incomplete observations [1]. It builds upon the solution of the initial value problem describing the time evolution of the material state, unlike the majority of data-driven approaches for constitutive modelling that require large data of increments of state variables. Numerical benchmarks showcase the benefits and accuracy of the proposed methodology. First, it removes the need of ``measuring'' state variables which cannot truly be measured or retrieved from real experiments (such as the elastic, or, equivalently, the plastic strain). Second, the approach demonstrates ability to learn accurate and robust constitutive models even in presence of generally complex model materials, and given only sparse sampling and corrupted measurement data. The newly developed formulation of neural integration for constitutive equations represents an important step to overcome the issues associated with data scarcity. Applications of the presented methodology in presence of real, experimental observations should be the ultimate objective of future works.