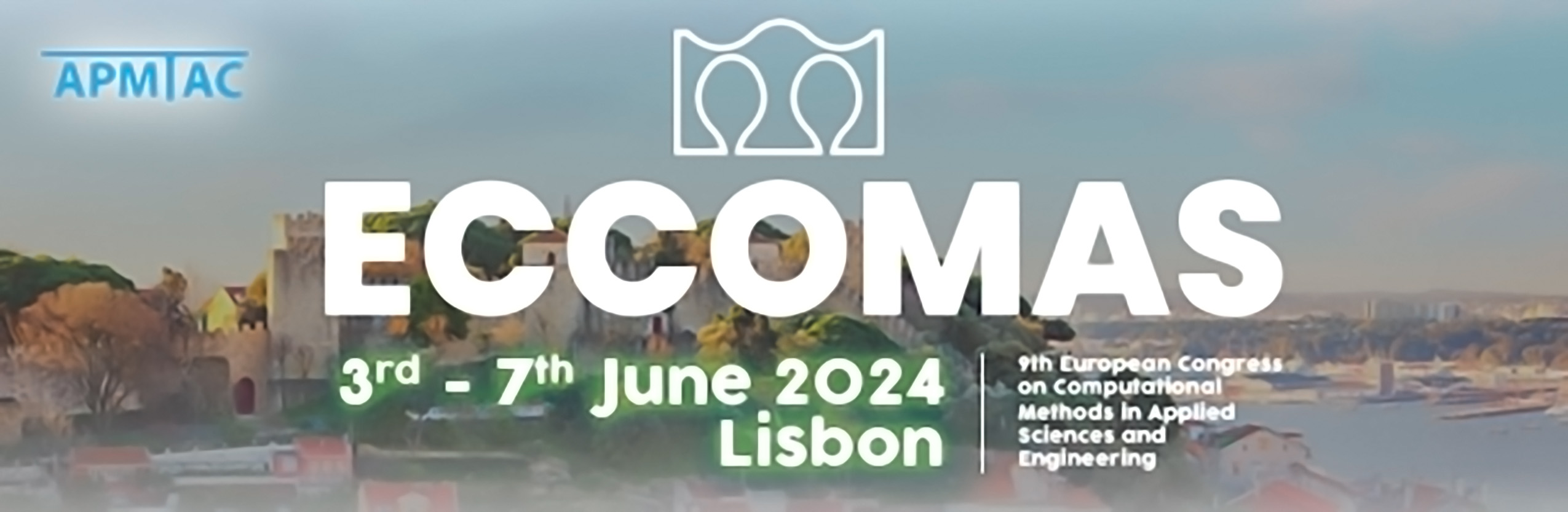
Integrating Curriculum Learning into hybrid modeling for improved predictive maintenance
Please login to view abstract download link
The state of health of a system can be verified using monitoring data from sensors. To process this data, machine learning methods, particularly the Long Short-Term Memory (LSTM) neural network, have garnered significant attention in the maintenance domain for their adaptability. While physics-based models are commonly employed to describe system health evolution, a comprehensive physical model for complex systems is often unavailable. This study proposes a hybrid model, combining both approaches for a balance between process accuracy and model adaptability. Initially applied to diagnose faults in an electric motor, the method is refined to predict future failures of motor components. The main contribution involves integrating an incremental learning strategy onto the hybrid model based on diverse known usage profiles, termed Curriculum Learning. This approach prioritizes the order of learning instances, demonstrating that restarting learning for new usage conditions is unnecessary. The methodology showcases the benefit of initially learning easy instances, aiding in the subsequent understanding of more complex instances while ensuring model stability. Upstream, a preliminary signal processing algorithm identifies the most influential profiles to build initial input data bases. The synthetic usage profiles thus obtained, characterized by type and features, undergo classification based on a customized complexity function and are progressively injected as training data. Preserving constitutional elements at each learning stage enables a comparison of neural structures, with curriculum-integrated structures outperforming those without in detecting system failures. This work contributes to a generalizable methodology, enhancing predictive capabilities of the hybrid model under new extrapolated usage conditions. This strategy also accelerates model convergence during the learning phase, thereby continuously improving model robustness. In fact, the method construction ensures convergence control upon the arrival of new, unknown data batches. When initial databases are insufficient for new usage conditions, supplementation with secondary databases prompts a learning process restart, aligning with fine-tuning methods in class-incremental learning. Therefore, the presented methodology provides insights into the interoperability of predictive models when integrating them into a new system or addressing other challenges.