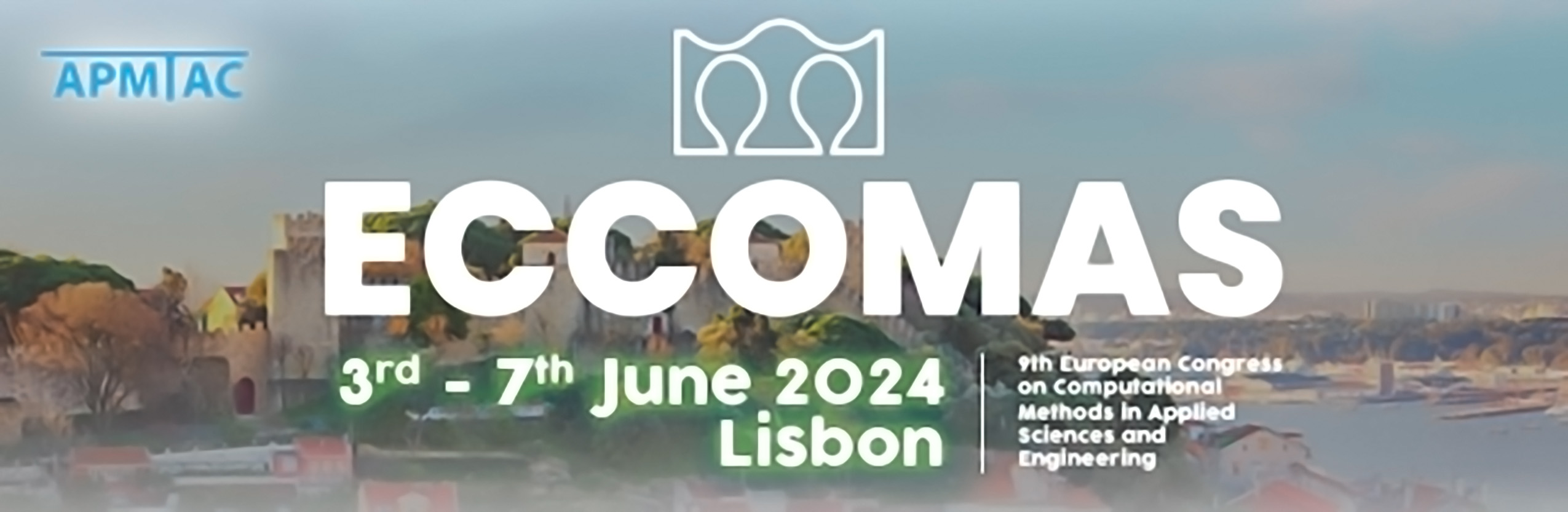
A non-intrusive mesh-free surrogate model to address geometrical variability in patient-specific hemodynamics
Please login to view abstract download link
Reduced order models (ROMs) offer precise and cost-effective approximations of patient-specific hemodynamics. Nonetheless, they often face challenges in handling geometrical variability. To address this issue, we propose to use the Universal Solution Manifold Network (USM-Net), a data-driven non-intrusive mesh-free surrogate solver for parametrized PDEs. To efficiently deal with patient-specific vessels, we exploit latent encodings and diffeomorphic mappings to a reference shape. The former offer a compact representation of the geometries, that can be seamlessly provided in input to the model. The latter, instead, help USM-Net in learning point-to-point correspondences, enhancing its predictive power at the testing stage. These two elements are in fact inherently related, since geometry deformations are obtained via a pre-trained conditioned neural field (called N-ODE LDDMM), where the embeddings act as conditioning variables within an auto-decoder structure. The patient-to-reference map invertibility is guaranteed by the use of a Neural ODE (N-ODE), which simultaneously learns and integrates the geometric flow equation stemming from the LDDMM framework. Finally, we present numerical tests on a cohort of 20 healthy aortic shapes, all featuring the same topology. The results show that N-ODE LDDMM is capable of learning accurate mappings to a reference shape, while USM-Net yields rather precise and yet cheap approximations of the velocity field.