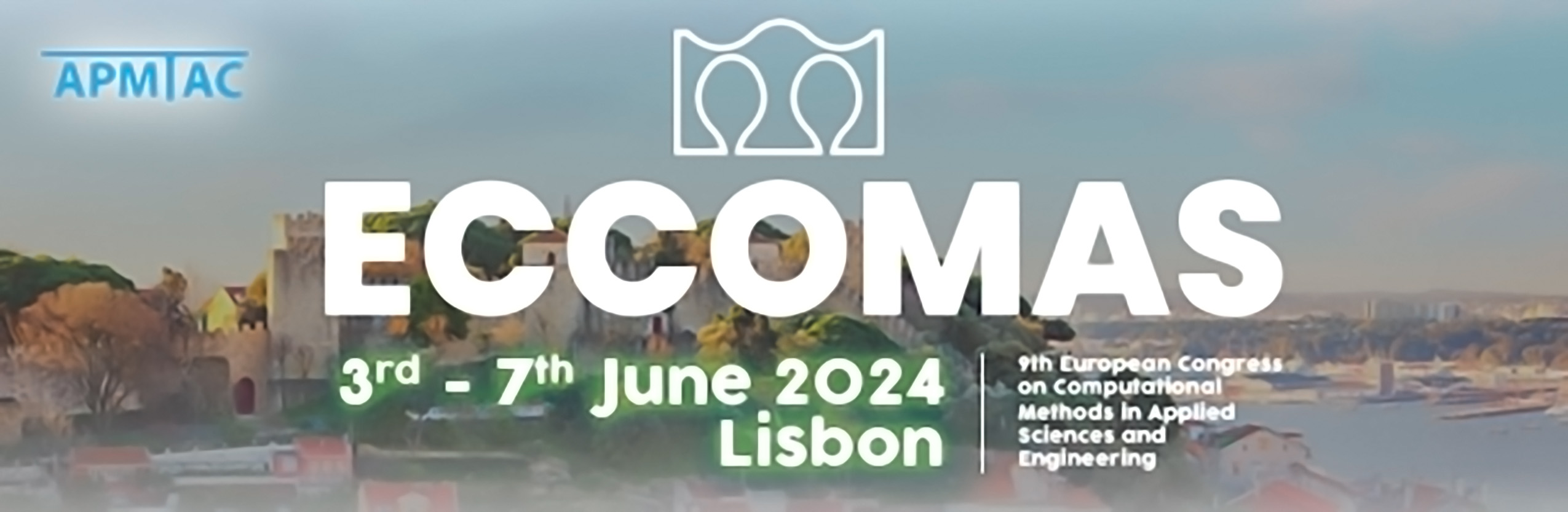
Real-Time Optimal Control of Parametrized Systems by Deep Learning-Based Reduced Order Models
Please login to view abstract download link
Many optimal control problems require suitable strategies in order to steer instantaneously the considered dynamics. Moreover, the control action needs to be updated whenever the underlying scenario undergoes variations, as often happens in applications. Full-order models based on, e.g., Finite Element Method, are not suitable for these settings due to the computational burden. In addition, conventional reduced order modeling techniques, such as the Reduced Basis method, are linear, intrusive and not efficient in addressing nonlinear time-dependent dynamics. We thus propose a nonlinear, non-intrusive technique based on Deep Learning-based Reduced Order Models (DL-ROMs) to control rapidly parametrized PDEs under different scenarios. Both low-dimensional and distributed control actions can be considered. In the former case, the physical constraint is replaced by a DL-ROM to speedup optimization. In the latter, optimal full-order snapshots are generated and reduced by deep autoencoders, while neural networks are exploited to learn the parameter-to-solution map. After data generation, dimensionality reduction and neural networks training in an offline phase, optimal control strategies can be rapidly retrieved online for all the scenarios of interest. The speedup and the high accuracy of the proposed approach have been assessed on different PDE-constrained optimization problems, ranging from diffusion-advection PDEs to unsteady Navier-Stokes equations.