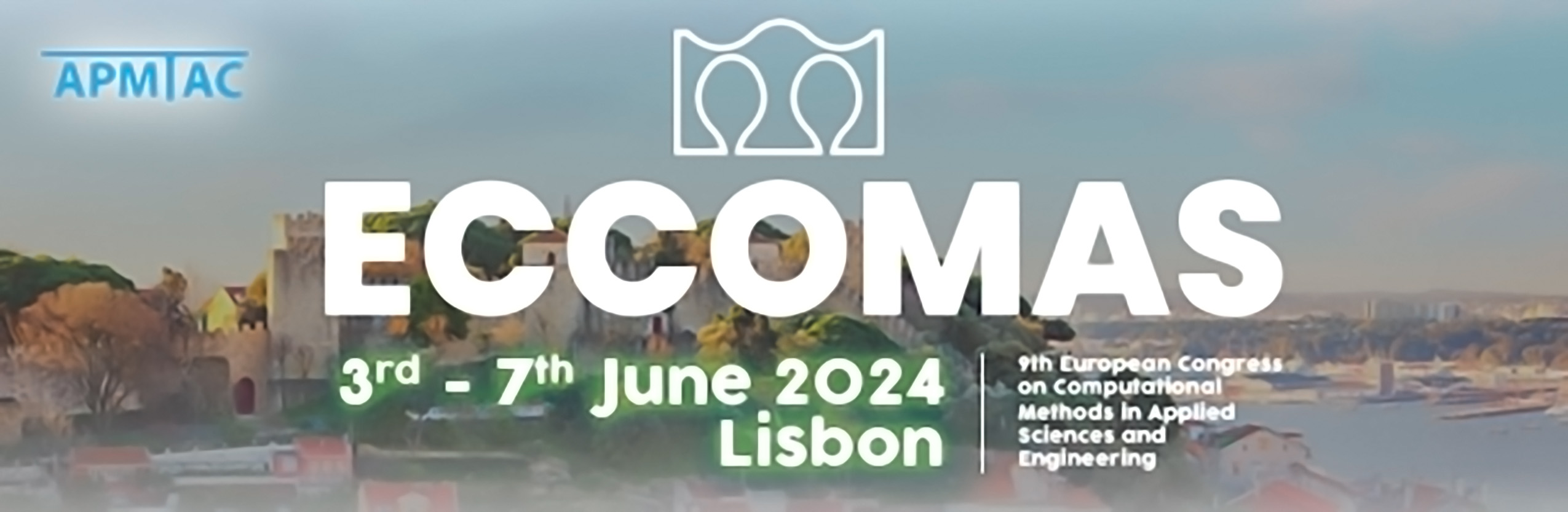
Efficient Active Learning for Sparse Gaussian Process Classifiers in SHM
Please login to view abstract download link
At the centre of many tasks within data-based Structural Health Monitoring (SHM) is the need to solve a classification problem, that is to automatically label data on the basis of observing certain features. However, there is a challenge in collecting comprehensive labelled datasets with which to construct these classifiers. There can be scenarios where data are available but assigning labels, requiring expert intervention, is expensive. This expense motivates labelling the minimum number of data to build a suitable classifier. Active Learning methods seek to achieve this by selecting and proposing the most informative data to label according to the output of some acquisition function. This work presents an efficient acquisition function for classification of SHM data in an active manner with the inclusion of uncertainty via a Gaussian Process Classifier (GPC). Many acquisition functions in the literature will target areas of high uncertainty in the latent space of the classifier, however this ignores that uncertainty is primarily important close to the decision boundary. This work proposes an acquisition function that specifically targets uncertainty over the decision boundary. Empirical results over synthetic and real world data show that the proposed acquisition function significantly minimise unnecessary data labelling --- hence cost. After the new data has been labelled, the model must be updated to incorporate this new data. Retraining the classifier is highly inefficient, so this work discusses two ways in which the updated posterior can be computed while avoiding heavy re-training over the updated data and also retaining sparsity. This is especially important given that sparsity is one of the few ways Gaussian Processes can be used in larger data environments. The effectiveness of this active GPC approach will be shown on an SHM example, motivating the use of the technique in the context of monitoring.