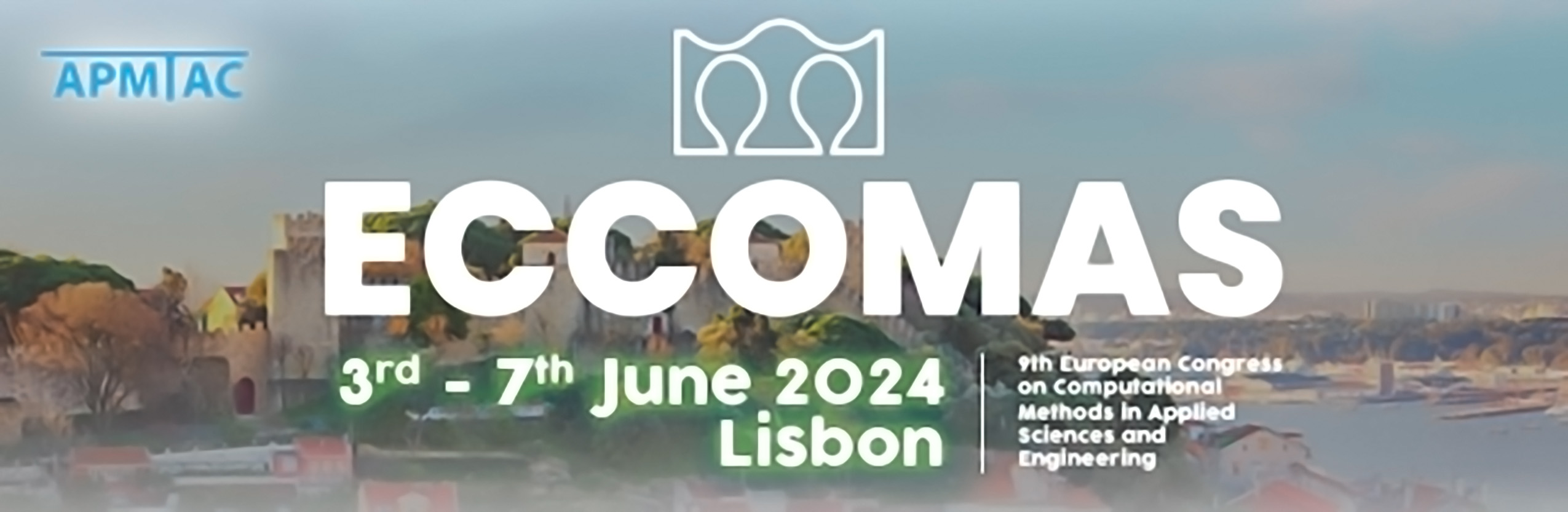
Enhancing bayesian model updating via learnable mappings with application to structural health monitoring
Please login to view abstract download link
In this talk, we present a new way to enhance stochastic approaches to structural health monitoring through the use of a learnable feature extractor and a feature-oriented surrogate model, both based on deep neural networks. These two data-driven models work in tandem to evaluate a likelihood function within a Markov chain Monte Carlo sampling algorithm. The feature extractor maps sensor recordings onto a low-dimensional metric space that encapsulates the sensitivity to structural health parameters. On the other hand, the surrogate model maps the structural health parameters onto their feature description. The procedure enables the updating of beliefs about the structural health parameters, effectively replacing the need for a computationally expensive finite element model. During a preliminary offline phase, we generate a labeled dataset to train both the feature extractor and the surrogate model. Within a simulation-based framework, training vibration responses are efficiently generated by means of a multi-fidelity surrogate modeling strategy. This multi-fidelity strategy exploits model order reduction and deep learning to speed up the data generation phase while preserving the damage-sensitivity of the approximated signals. The proposed strategy is assessed through synthetic test cases, demonstrating impressive results in terms of accuracy of the estimated quantities and computational efficiency. Starting from the results obtained using numerically generated data, we discuss some open issues related with damage localization and quantification in structural health monitoring focusing on the combined use of deep learning, reduced order modeling, and uncertainty quantification methods, and explore some general facts that can be of interest in other contexts as well.