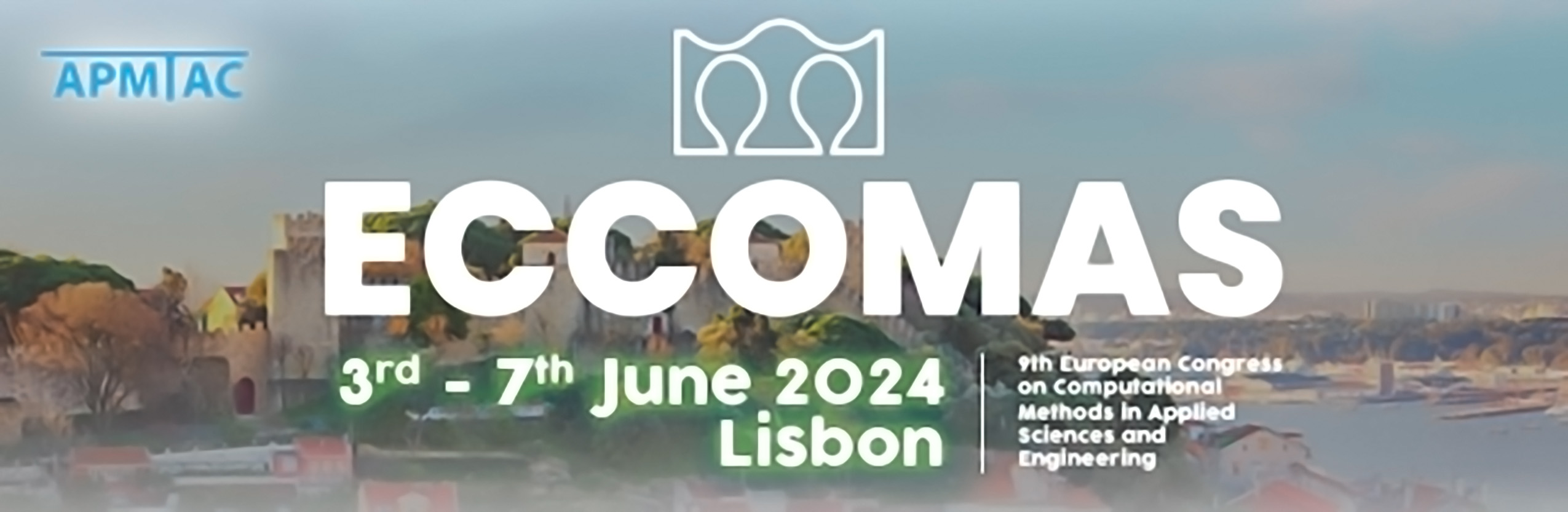
Microstructural Characterization in Aerogel Modeling through Deep Symbolic Regression
Please login to view abstract download link
Aerogels are known for their exceptional properties such as low density and high surface area, as well as for their exceptional thermal insulation properties. Two different modeling approaches, the diffusion limited cluster-cluster aggregation (DLCA) method for silica aerogels and the Laguerre-Voronoi tessellation for nano porous open-cell aerogels, can be used to study the influence of their fractal morphology and network structure on the mechanical behavior. In this contribution, we present a novel method to improve the microstructural characterization in aerogel modeling using deep symbolic regression (DSR). Symbolic regression is a data-driven method that aims to identify an algebraic expression that best describes the relationship between input variables and a given output. Unlike neural networks, DSR provides a white box model that can be interpreted even for complex systems such as those found in aerogel modeling. In this work, insights are gained to better understand the intricate relationships between input parameters and the resulting structures in aerogel modeling. The influence of e.g. particle radius or pore size distributions is investigated to identify material models that incorporate their effects directly within a strain energy function.