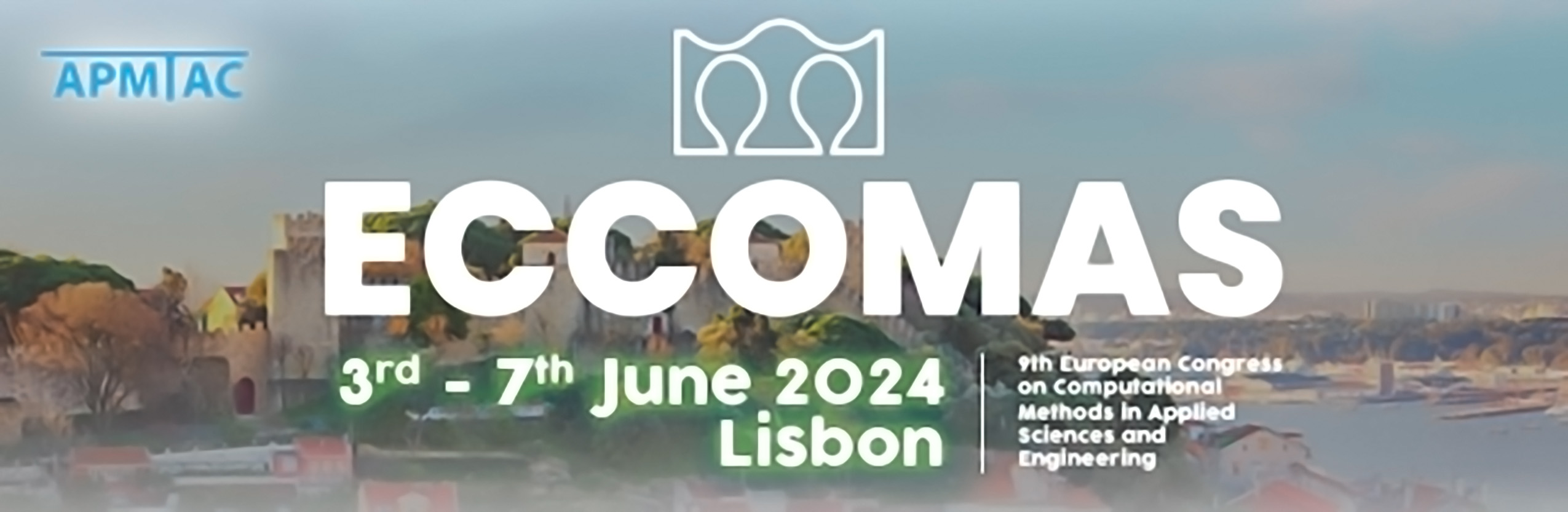
Enhancing Coronary Artery Segmentation for Hemodynamic Simulations: A Semi-Automatic Approach
Please login to view abstract download link
Coronary artery disease (CAD) characterized by the narrowing of coronary arteries, known as a stenosis, remains a leading cause of mortality worldwide. The growing of CAD is emphasized by the increase in global death counts from 12.4 million in 1990 to 19.8 million in 2022 [1]. Accurate assessment of stenosis and blood flow in these sections are important for effective treatment planning. In this regard, Fractional Flow Reserve (FFR) analysis under hyperemia conditions is a key metric in assessing the physiological impact of stenosis by measuring the pressure differences across a stenotic section. Maximal hyperemia is essential for achieving a correlation between coronary pressure and blood flow [3]. This is currently performed through an invasive procedure. However, advances in cardiac imaging, e.g., coronary angiography computed tomography (CACT) have increased the potential to perform this assessment noninvasively by extracting the anatomy of interest from the images and performing hemodynamic simulations. To this end, the first step it to identify and segment the coronary tree, from the CT images, to create the models for simulation. However, traditional methods of manual artery segmentation and 3D model preparation for hemodynamic simulations are time-consuming and often affected by human error [2]. This not only affects the precision of the simulations but also delays the diagnosis. Our work proposes a novel semi-automatic method for coronary artery segmentation and creation of a 3D hyperemia model significantly reducing the time and manual effort required by the manual procedure. This method relies on computational methods implemented in Python due to its versatility and vast range of libraries for medical imaging. By striking a balance between automated efficiency and manual intervention, reliability is enhanced compared with fully automatic methods. Preliminary assessment shows promising results and the method's accuracy is currently being validated considering real patient data. The proposed method has the potential to speed the research on hemodynamic simulation, FFR in particular, constituting an important step towards its translation into application scenarios, e.g., by physicians.