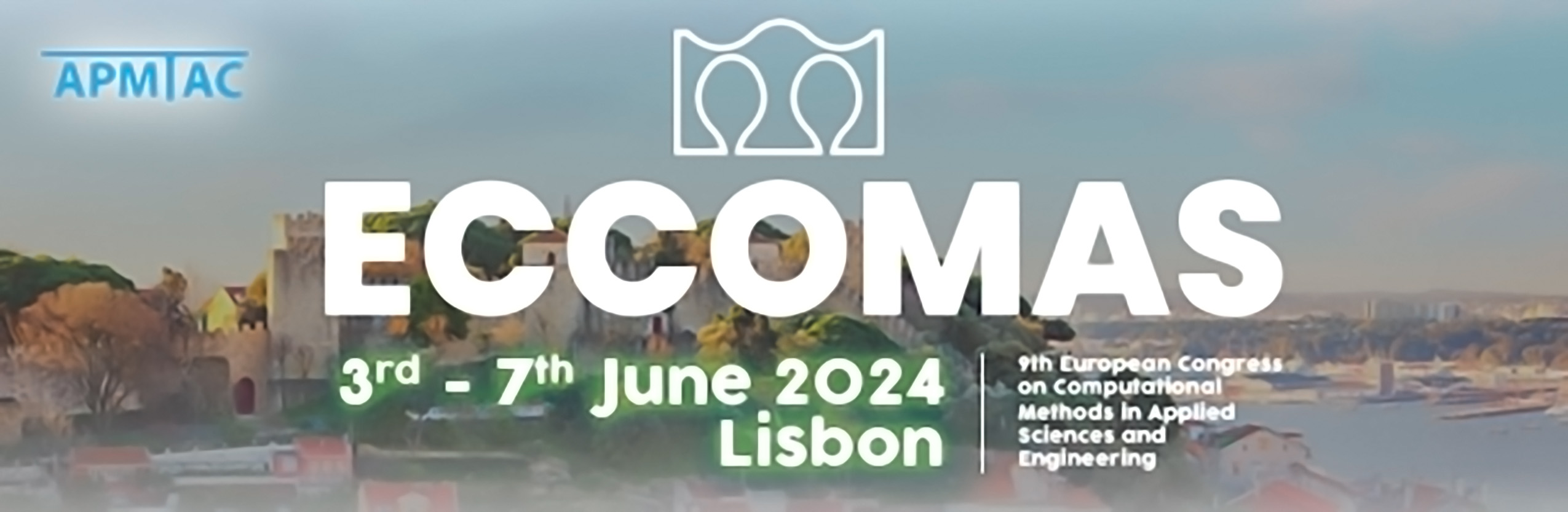
Prediction Accuracy of Entropy Generation Mechanisms in a Compressor Cascade
Please login to view abstract download link
Accurate numerical prediction of flow physics and incurred loss mechanisms is necessary for further improvements in aircraft engine efficiency. Due to the cancellation of errors, RANS calculations show sufficient accuracy with regard to integral values. However, local deficits in prediction accuracy in highly complex transonic compressor flows comprising, e.g., shock-boundary layer interactions are present. The application of an entropy generation based loss analysis highlighted shortcomings in turbulence-related mechanisms. To help understand the origin of these shortcomings, in this paper, a less complex test case consisting of a NACA 65 compressor cascade is investigated. Even for this test case without shock-boundary layer interaction but a small separation region, RANS calculations show local deficits compared to scale-resolving LES results. The entropy generation in the suction side separation region and the wake significantly differs from high-fidelity results, especially in an off-design condition. However, to this day, LES is not feasible in industrial design iterations due to high computational effort. To overcome the incurred errors in RANS, hybrid RANS/LES methods locally resolving part of the turbulent spectrum are performed while only moderately increasing the computational effort. Hence, hybrid methods increase prediction accuracy in detached flow regions and large-scale structures in the wake, where turbulence-related entropy generation dominates. The investigation of different mesh resolutions and model combinations enables the determination of the sensitivity of hybrid methods, thereby providing a comparison between increased prediction accuracy and required increased computational effort. The application of these methods shows satisfactory improvements in loss prediction, thus partially closing the gap between RANS and LES calculations.