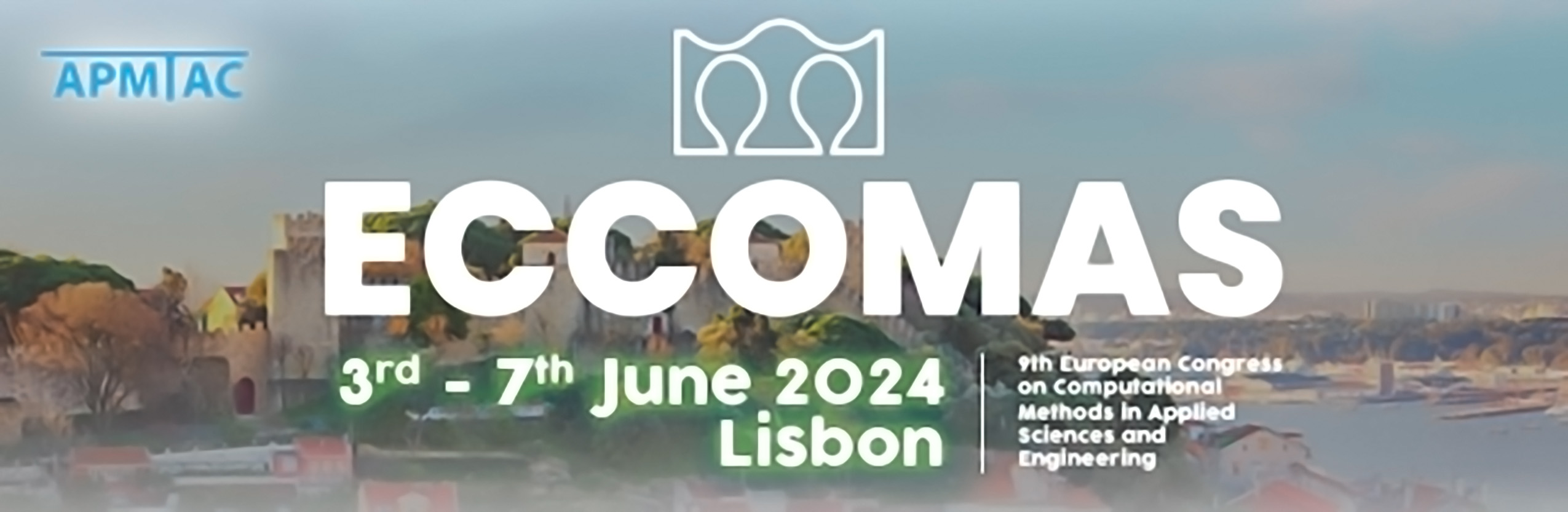
Clustered Active Subspaces for Surrogate-Based Aerodynamic Shape Optimisation
Please login to view abstract download link
Surrogate-based optimisation in design spaces exceeding several tens of parameters is numerically intractable yet more and more frequent. Dimension reduction is a potent answer to this problem. However, typical dimension reduction methods seek to identify global trends over the entire design space. We propose to use a combination of Active Subspaces in subregions of the design space, where their use of the objective function’s gradients will exploit local information to discover specific trends in the objective function. Local surrogate models are then constructed in the subspaces, and recombined to form a global surrogate model, which we can then exploit for optimisation. The partitioning of the input space is done through a model based Gaussian Mixture Model clustering, where the authors assume the distribution of the joint inputs/outputs to be a mixture of Gaussians. After labelling the points with GMM, we propose to train a supervised Support Vector Classifier. This strategy scales better with high-dimensional parameter spaces and yields an explicit analytical expression of the clusters’ boundaries, from which we can formulate an improved recombination strategy with overlapping between the surrogate models. The use of overlapping increases prediction accuracy at the boundaries between clusters, an improvement over recombination methods typically found in the literature.