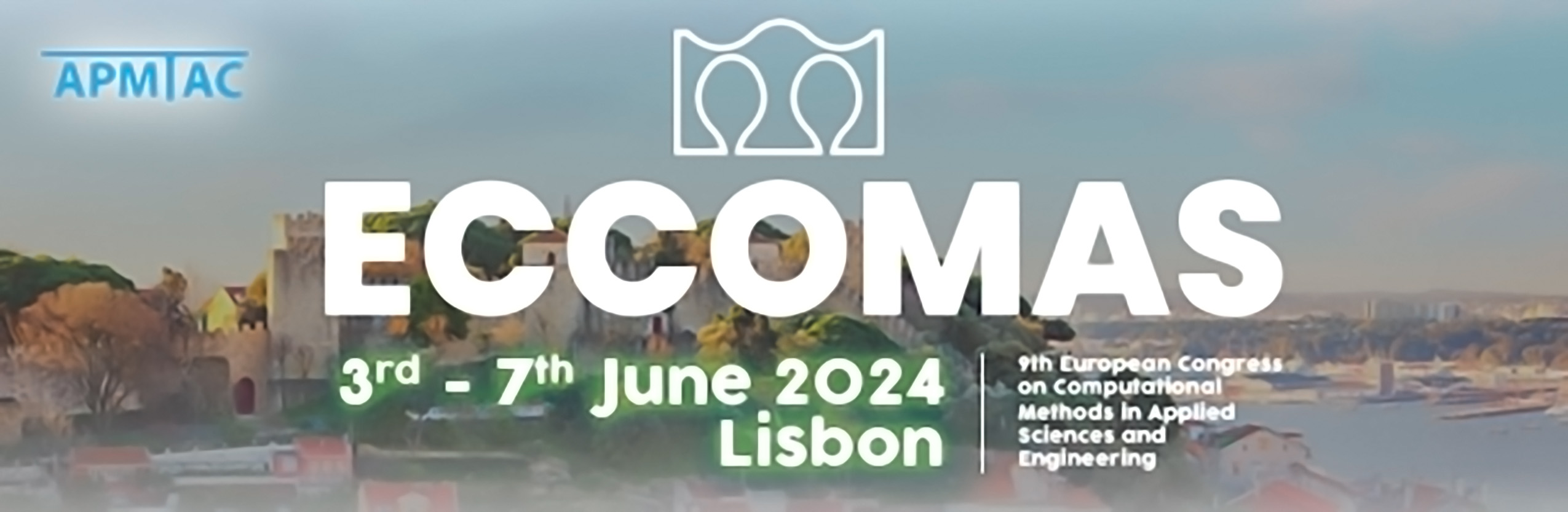
Predicting Coronary Plaque Vulnerability through a Radiomics-Biomechanics Approach
Please login to view abstract download link
Detecting coronary vulnerable plaques is a crucial aspect of cardiovascular medicine, with the potential to prevent major adverse cardiac events and decrease cardiovascular mortality. While various biomarkers for plaque vulnerability (e.g., image, biomechanical, and omics-based) have been suggested, accurate patient stratification remains elusive, and comprehensive approaches integrating multiple markers are lacking. This study proposes a novel method for the assessment of vulnerable plaques, by integrating radiomics (i.e., the extraction of quantitative features from radiological images) and biomechanical markers through machine learning (ML). The dataset included 35 patients (with 7 vulnerable and 36 non vulnerable arteries) who underwent coronary computed tomography angiography (CCTA). The study employed a leave-one-out cross-validation scheme for feature selection, ML model training, and testing. Radiomics and radiomics-biomechanics classifiers demonstrated comparable stratification performances. The best radiomic classifier achieved a balanced accuracy of 0.83, correctly identifying all 7 vulnerable arteries. The best radiomics-biomechanics classifier showed a balanced accuracy of 0.82, identifying 6 out of 7 vulnerable arteries. In conclusion, the study demonstrates the potentialities of the proposed radiomics and radiomics-biomechanics approaches for vulnerable plaque prediction.