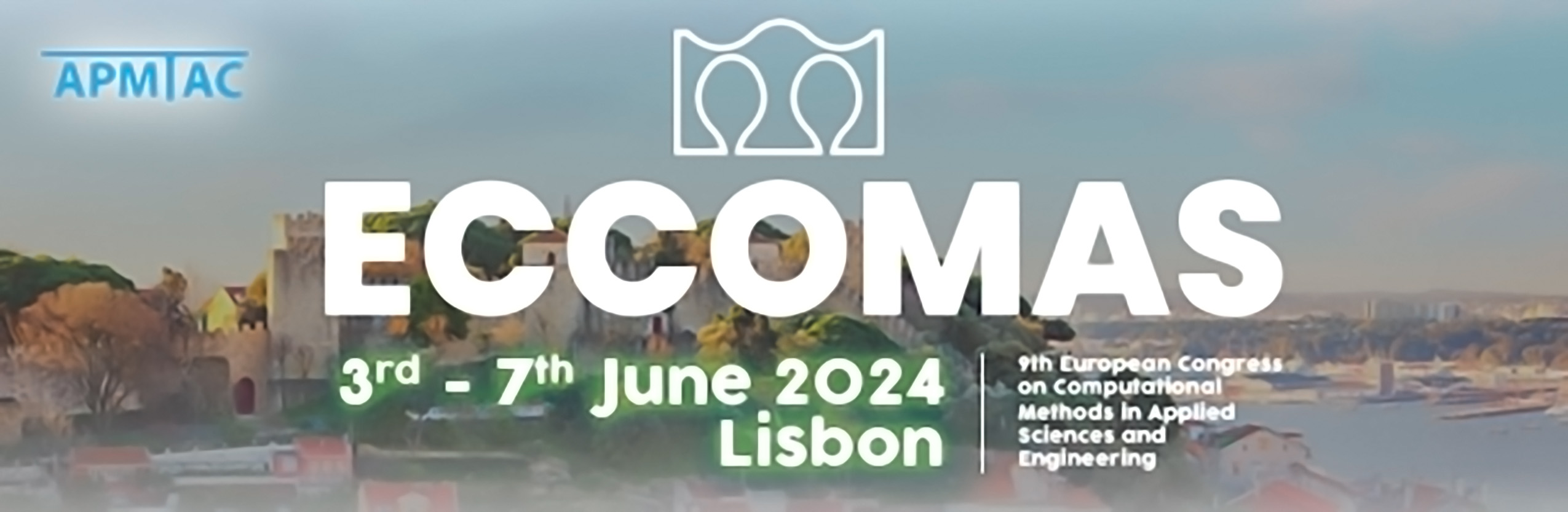
Multiscale Numerical Methods and their Interplay with Artificial Intelligence
Please login to view abstract download link
The tremendous development of massively parallel architectures has led to a review of computational simulators, which must incorporate asynchronous and communication-avoiding algorithms. In this scenario, where accuracy and robustness remain fundamental properties but algorithms must take full advantage of new architectures, multiscale numerical methods built on the ``divide and conquer'' philosophy have become an attractive option. Among the various options, we are interested in the family of multiscale methods called Multi-scale Hybrid-Mixed Method [1,2] (MHM, for short). In this work, we bring some ideas [3] and discussions about how the MHM methods and machine learning techniques (operator learning, physics-informed neural networks, etc.) may benefit from each other, rendering innovative methods with a drastic decrease in computational cost without loss of numerical precision.