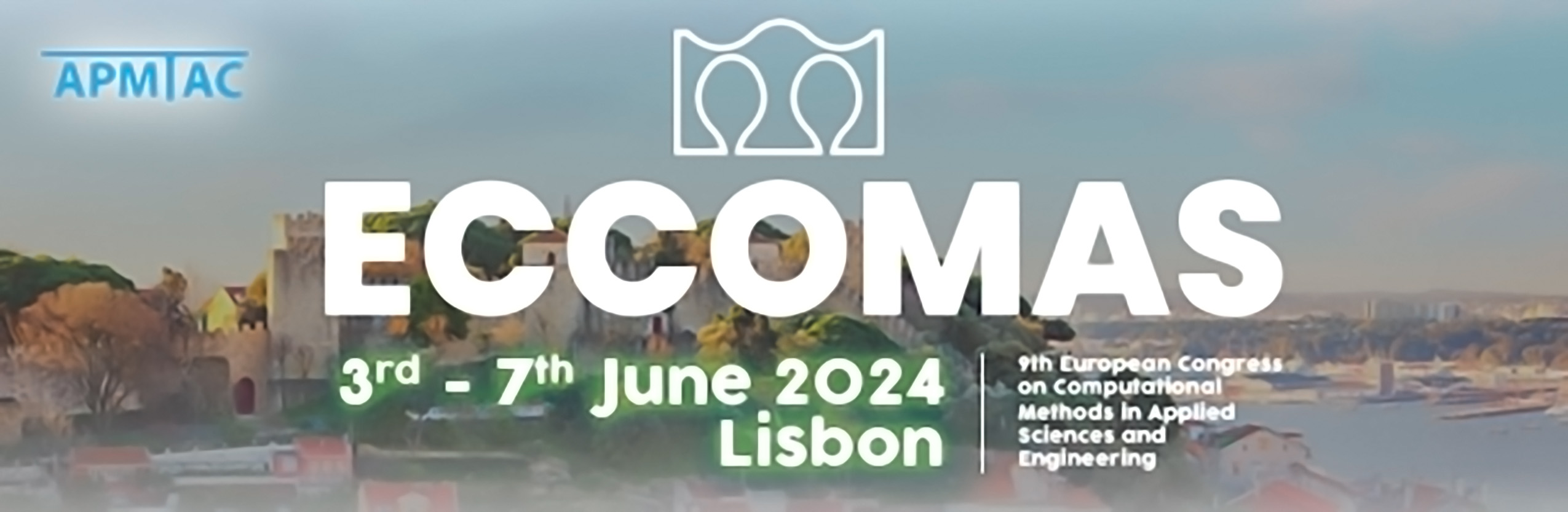
Towards ExaScale Training: Domain Decomposition Methods for the Efficient Training of Neural Networks
Please login to view abstract download link
The efficient training of nowadays large networks requires efficient, robust, and parallel training methods. Inspired by subspace correction methods we present globally convergent methods, which allow for the accurate, efficient and parallel training of neural networks. We discuss the general principles underlying their construction, i.e. decompositions in data and weights; furthermore, we provide an abstract methodological framework for parallel training. Eventually, we discuss how to avoid time-consuming hyper-parameter search by employing suitable convergence control strategies. Numerical examples illustrating our findings are presented.