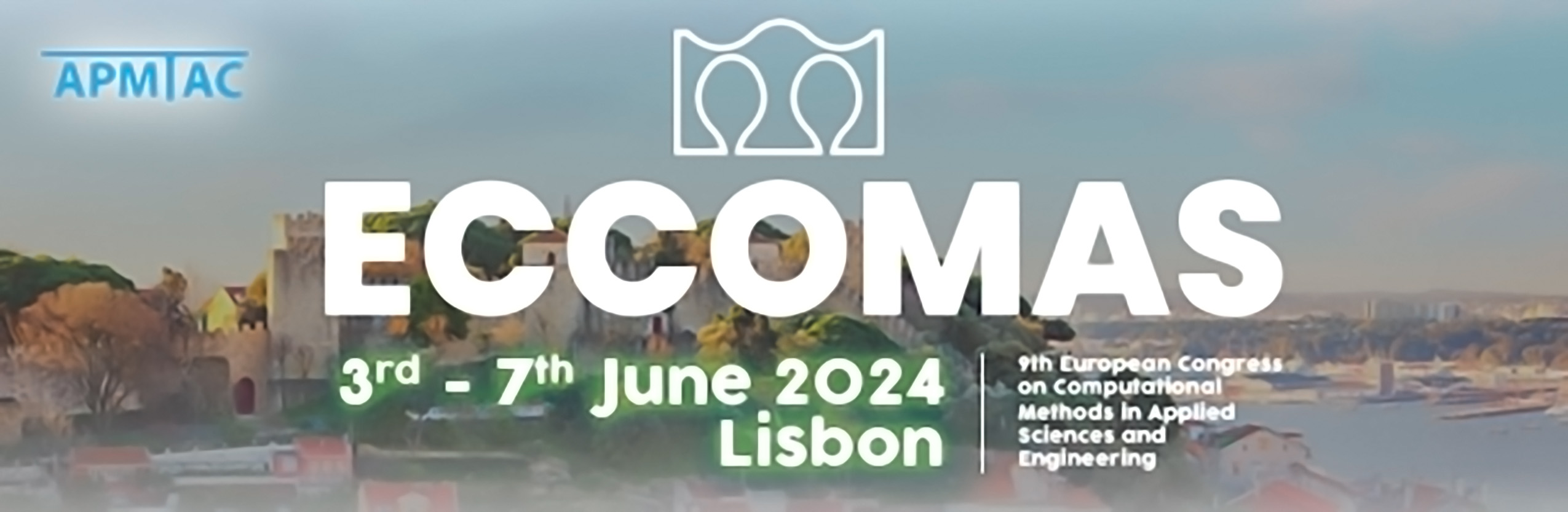
Multi-Objective Optimization in Physics Informed Neural Networks
Please login to view abstract download link
In the training of physics-informed neural networks (PINNs), multiple objective func- tions must be minimized. PINNs always involve the squared residual of the partial- differential equation, accounting for the physics. Moreover, a loss for the initial and/or boundary conditions, as well as other potential data points, can be incorporated. For multi-domain or multi-physics problems, each subproblem, as well as the coupling terms, may also lead to additional objective functions. In practice, the optimization problem becomes more difficult the more (potentially conflicting) objective functions are added. In this talk, we will discuss two different approaches to improve the training in the presence of multiple objective functions. On the one hand, we will discuss the use of multi-objective optimization (MOO) to optimize objectives that are conflicting in nature. On the other hand, we will introduce a systematic framework to treat initial and boundary condi- tions as well as coupling conditions for multi-domain and multi-physics problems by hard constraints. We will systematically test the performance of soft and hard constraints, as well as combinations thereof, for a classical domain decomposition of a transmission problem into two subdomains