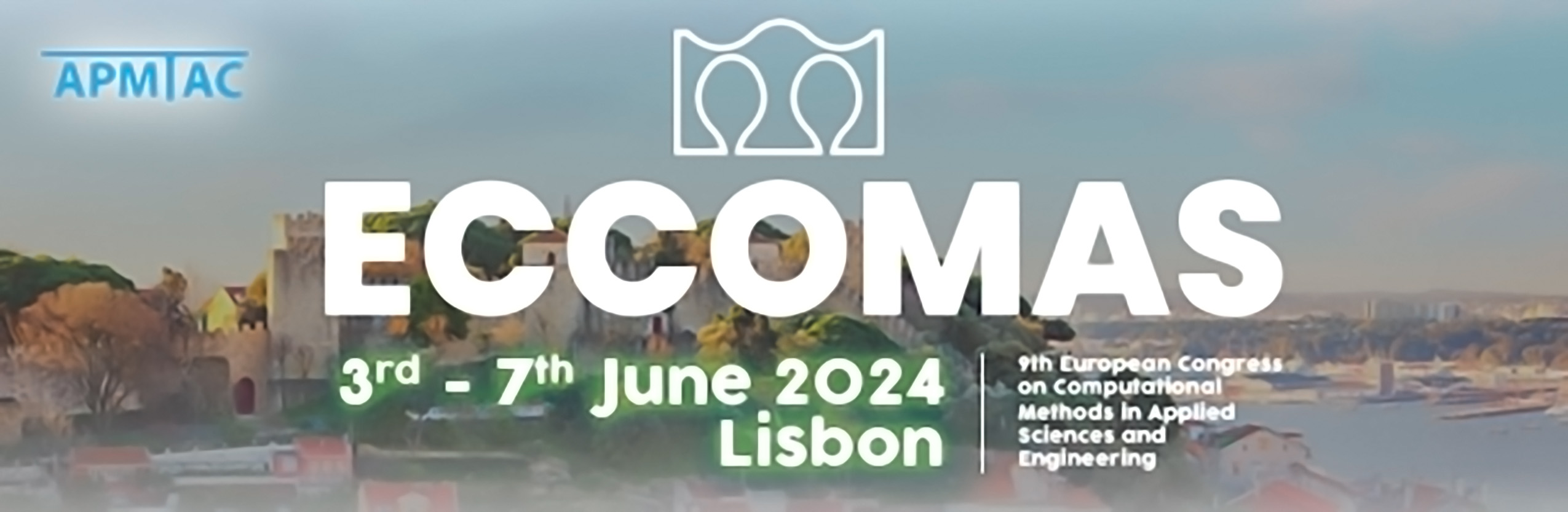
Data-driven Modeling of Multi-physics Coastal Hydrodynamics using a Deep Neural Operator Framework
Please login to view abstract download link
High-fidelity numerical models are commonly used to simulate physical phenomena in coastal and near-shore regions that involve nonlinear interactions between wave hydrodynamics, meteorological, and hydrological phenomena. Despite the tremendous progress in computational resources and methodologies, these high-fidelity models are still computationally expensive and require a significant amount of domain expertise. Moreover, in addition to the high computational cost, the challenges of capturing nonlinear spatial features using unstructured meshes across coupled models and the adoption of various empirical approximations render most of the existing high-fidelity, coupled wave-circulation models ineffective for use in ensemble-based forecasting systems and coastal hazard assessment studies. In this talk, we will present a deep neural operator framework inspired by the deep operator network (DeepONet) philosophy that can generate fast and accurate real-time predictions of various parametrized scenarios arising in coastal flows. We will explore different model formulations and demonstrate their merits and drawbacks using both a benchmark analytical problem as well as a realistic example of 2D tide-driven flow in Shinnecock Inlet Bay, New York. Moreover, a typical design and development pipeline for a model problem will be presented to highlight how such a framework might fit as a general approach for reduced order modeling in multi-physics engineering applications.