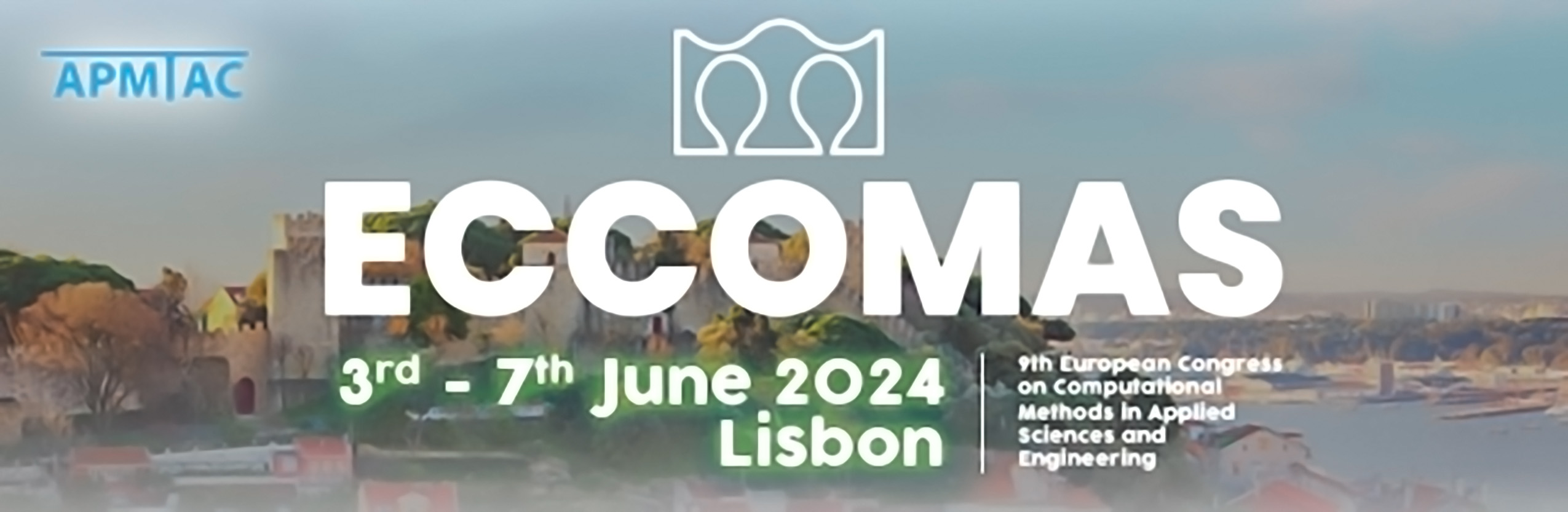
Turbidity Currents Simulations in Channels with Different Slopes Using ROM-NN Models
Please login to view abstract download link
Turbidity currents are a particle laden flow describing a complex natural phenomenon. They are turbulent density driven flows and a mechanism responsible for depositing sediments on a seabed. Their understanding may help geologists gain insights into reservoir formation. We present a numerical method applied to the simulation of turbidity currents in an Eulerian-Eulerian framework. The challenge is the high computational cost of evaluating the high-fidelity (HF) model. The present work aims to build a surrogate model to mitigate such drawback. It consists of a neural network that infers the concentration field of turbidity currents for a given time and specific parameter. The data for training this predictive model comes from simulations of the HF model for a set of values in the parameters space. The equations are spatially approximated via the stabilized finite element technique using RBVMS formulation. We consider the lock-exchange configuration for several channel slopes (an essential parameter controlling the flow). The HF solution for each angle is generated for several time instants. A snapshot matrix containing the concentration values at the mesh nodes is constructed for each angle. For the training process, a large snapshot matrix is generated by concatenating the previous snapshot matrices. We then consider two dimensionality reduction techniques that enable the use of the surrogate model. In the first approach, in the offline stage, we employ a linear reduction via Singular Value Decomposition (SVD) followed by the training of the surrogate model. In the online stage, we infer the values on a reduced basis and later return to the concentration values at the original space via the SVD. For the second approach, the offline stage uses the previous SVD and subsequently trains an autoencoder (for the nonlinear reduction), followed by the training of the surrogate model for the values at the latent space of the autoencoder. In the online stage, one infers the values at the autoencoder’s latent space, applies the trained decoder to return to the reduced basis, and again uses the SVD to return to the original concentration space. We test our approaches on a parameter value not present in the training set and compare their solutions and errors to the corresponding HF solution. We conclude the second approach produces solutions with lower errors than the first one, with similar computational costs in the online stage, although with higher training time.